Why Getting Data Labelling Right is Critical for Business Success

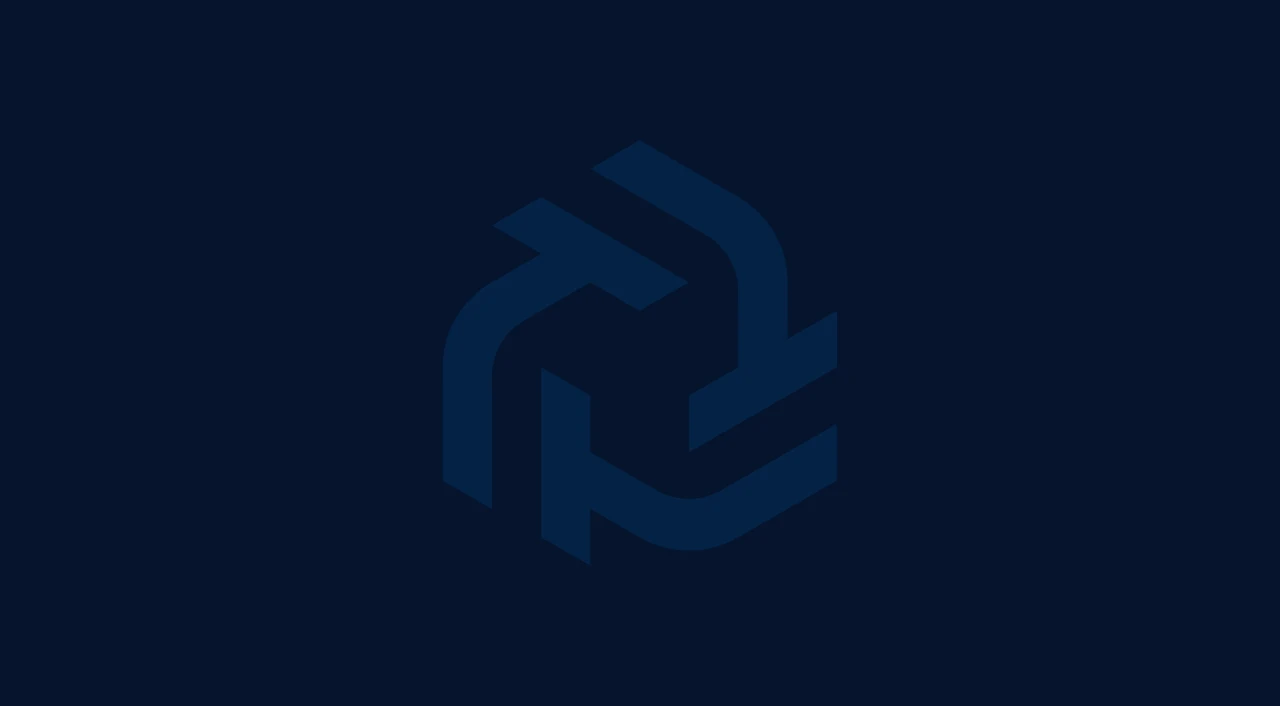
When you consider the pace at which organisations are looking to adopt artificial intelligence (AI) and reap its benefits, the focus is often initially on the end goal - deploying powerful models that can transform their operations. However, it’s the operational aspect itself that also needs consideration. An often-overlooked aspect that can make or break the success of AI initiatives is data labelling. In this article, I’ll explore why this often considered rudimentary, back-office element of LLM is actually the unsung hero of AI, as well as share cautionary tales of what can go wrong when it’s not properly considered, and provide practical advice on how businesses can navigate this critical process.
The power and pitfalls of LLMs
There’s no doubt that Large Language Models (LLMs) have demonstrated remarkable capabilities, from generating human-like text to solving complex problems. Their potential for businesses is vast, ranging from automating customer support to optimising supply chain operations. However, the limitations of foundational models for specific business use cases cannot be overlooked. Without proper fine-tuning and domain-specific data, LLMs can produce inaccurate or irrelevant results. A recent study from Oxford University exposed the dangers of inaccurate, or hallucinated, LLM responses, and this can often occur when applied to niche domains without adequate data labelling.
The data labelling dilemma
High-quality, domain-specific data is the key to unlocking the full potential of LLMs for businesses. However, building and managing data labelling teams presents a unique set of challenges. Ensuring data quality while meeting tight deadlines is a delicate balancing act that requires careful planning and execution. Labellers must possess domain expertise, consistency, and attention to detail – a combination that can be difficult to find and manage at scale. According to a survey by Cognilytica, 80% of the time spent on AI projects is dedicated to data preparation and labelling, highlighting the critical role of this process in the success of AI initiatives.
Real-world examples
At TextMine, we experienced the importance of effective data labelling firsthand while building our platform for extracting insights from PDFs. Our journey involved a trial-and-error process of designing data labelling workflows that could deliver high-quality results while keeping pace with our development timeline. We learned valuable lessons along the way, such as the importance of clear labelling guidelines, regular quality checks, and open communication channels for our labelling team. By implementing these best practices, we were able to improve the accuracy of our models by over 30% and reduce labelling time by 25%.
The consequences of improper data labelling can be severe, particularly in sensitive domains like legal and procurement verticals. For example, imagine a company implementing an AI system to automate the review of legal contracts. If the data labelling process fails to accurately identify and label key clauses, such as termination conditions or liability limitations, the AI model may overlook critical information or provide incorrect recommendations. This could lead to the company entering into unfavourable agreements or facing legal disputes due to misinterpreted contract terms. The fines associated with these mishaps when it comes to regulatory audits and compliance issues can be astronomical.
Similarly, let’s consider the procurement context, AI can be used to detect and prevent fraudulent activities, such as bid-rigging or supplier collusion. However, if the data labelling process does not correctly identify and label instances of fraud within the training data, the AI model may fail to recognise suspicious patterns or anomalies in real-world scenarios. This can result in fraudulent activities going undetected, leading to financial losses and reputational damage for the organisation.
These examples underscore the critical importance of accurate and comprehensive data labelling in AI development, particularly in sensitive domains like legal and procurement. The consequences of improper labelling can be severe, ranging from financial losses and legal liabilities to damaged reputations and eroded trust in AI systems. As businesses increasingly rely on AI to automate decision-making processes, ensuring the quality and integrity of the underlying training data through meticulous labelling practices becomes a non-negotiable priority.
Charting the course for effective data labelling
To navigate the data labelling process successfully, businesses must start by identifying and prioritising their labelling needs based on the specific requirements of their AI projects. This involves carefully evaluating the complexity of the task, the volume of data required, and the level of domain expertise needed. Once these factors are established, companies can develop a strategy for sourcing and managing high-quality data labellers. This may involve partnering with specialised data labelling services, recruiting in-house experts, or leveraging crowdsourcing platforms. The use of tools and technologies, such as data annotation software and quality control mechanisms, can help streamline the labelling process and ensure consistency across large datasets.
The future of data labelling: trends and predictions
As AI continues to evolve and permeate every industry, the demand for specialised data labelling services is expected to grow exponentially. The global data annotation tools market is projected to reach $15.2bn billion by 2032, growing at a CAGR of 6.5% from 2023 to 2032. Advances in AI and automation may help simplify certain aspects of the labelling process, but the need for human expertise and oversight will remain crucial. Businesses that stay ahead of the curve by adopting best practices and investing in data labelling will be well-positioned to gain a competitive edge in the AI landscape.
Data labelling may be the unsung hero of AI, but its critical role in the success of AI initiatives cannot be overstated. By prioritising and investing in this essential process, businesses can unlock the true potential of AI and drive transformative results. The path to effective data labelling requires careful planning, strategic execution, and a commitment to continuous improvement. As the potential and adoption of AI technology continues to evolve and accelerate, companies that master the art and science of data labelling will be the ones that shape the future of their industries and reap the rewards of AI-driven innovation.
About TextMine
TextMine is an easy-to-use data extraction tool for procurement, operations, and finance teams. TextMine encompasses 3 components: Legislate, Vault and Scribe. We’re on a mission to empower organisations to effortlessly extract data, manage version controls, and ensure consistency access across all departments. With our AI-driven platform, teams can effortlessly locate documents, collaborate seamlessly across departments, making the most of their business data.
Newsletter
Blog
Read more articles from the TextMine blog
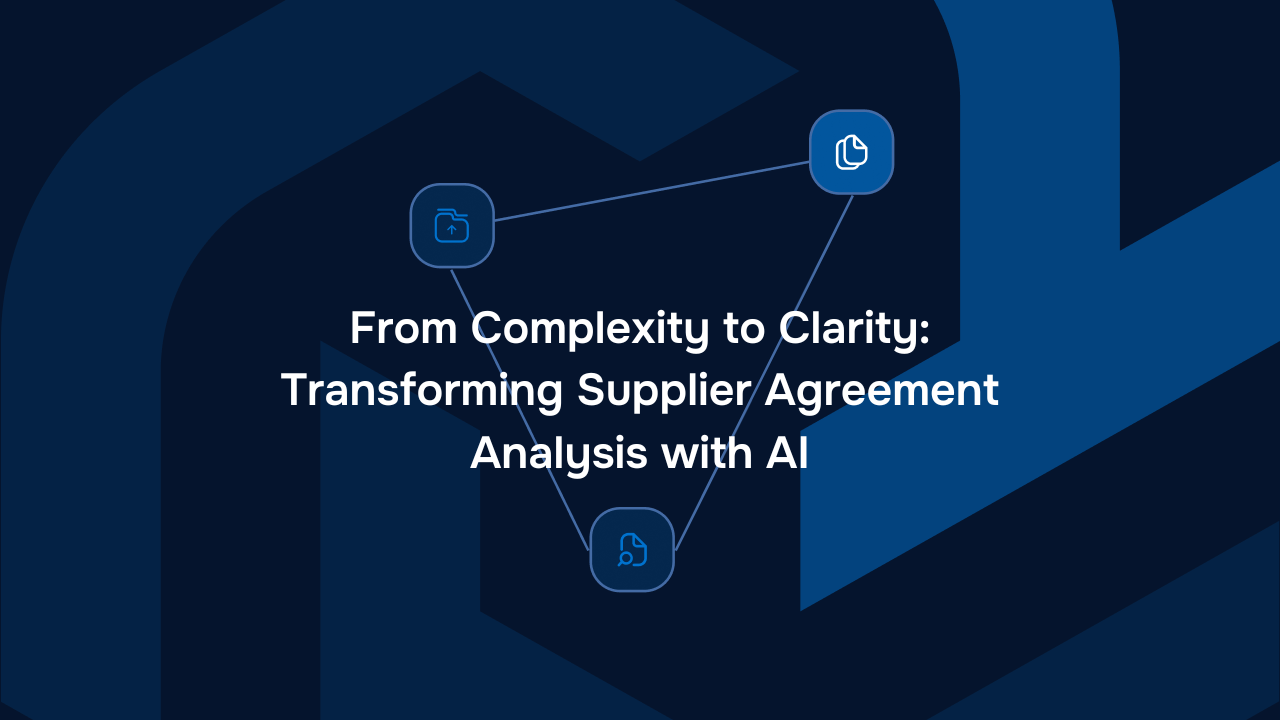
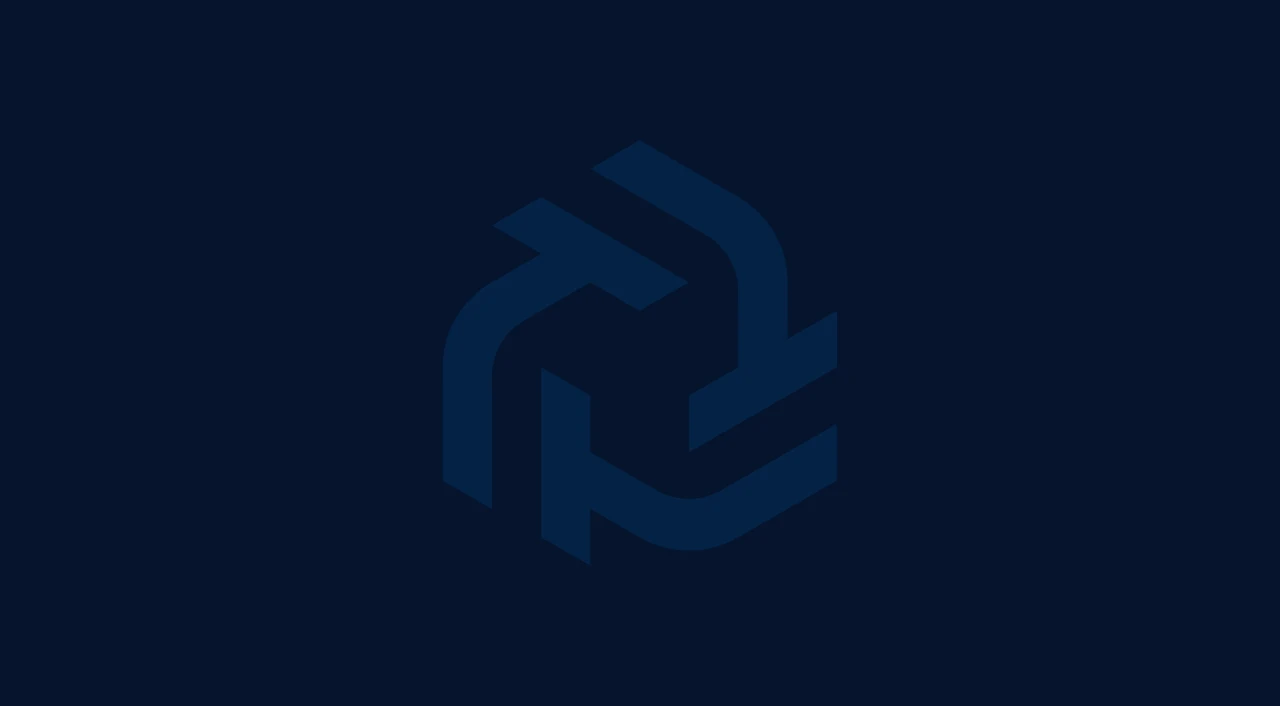
From Complexity to Clarity: Transforming Supplier Agreement Analysis with AI
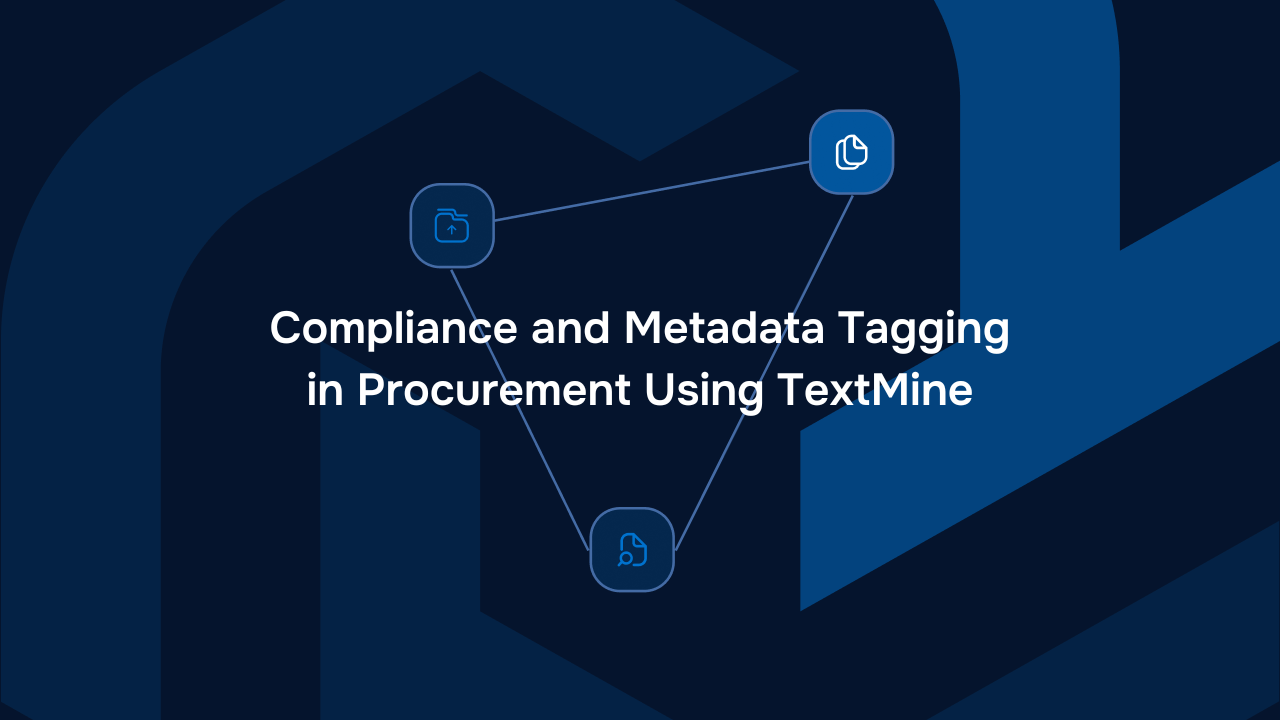
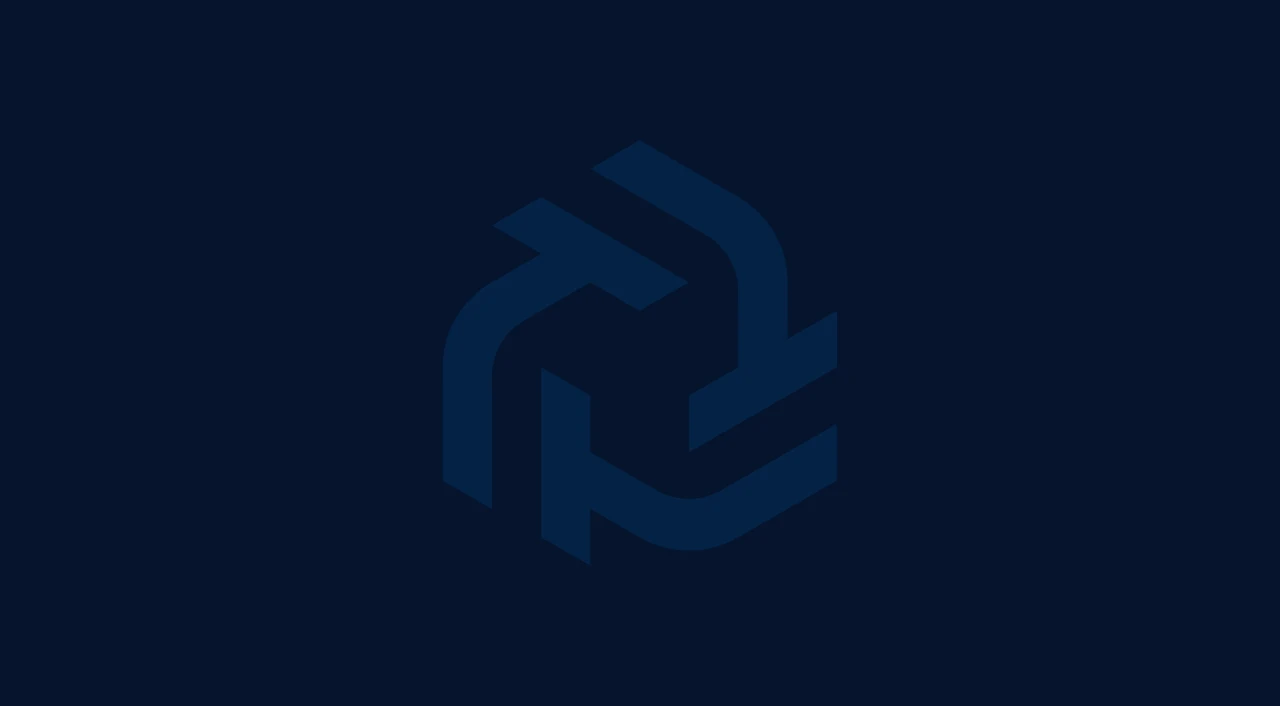
Compliance and Metadata Tagging in Procurement Using TextMine
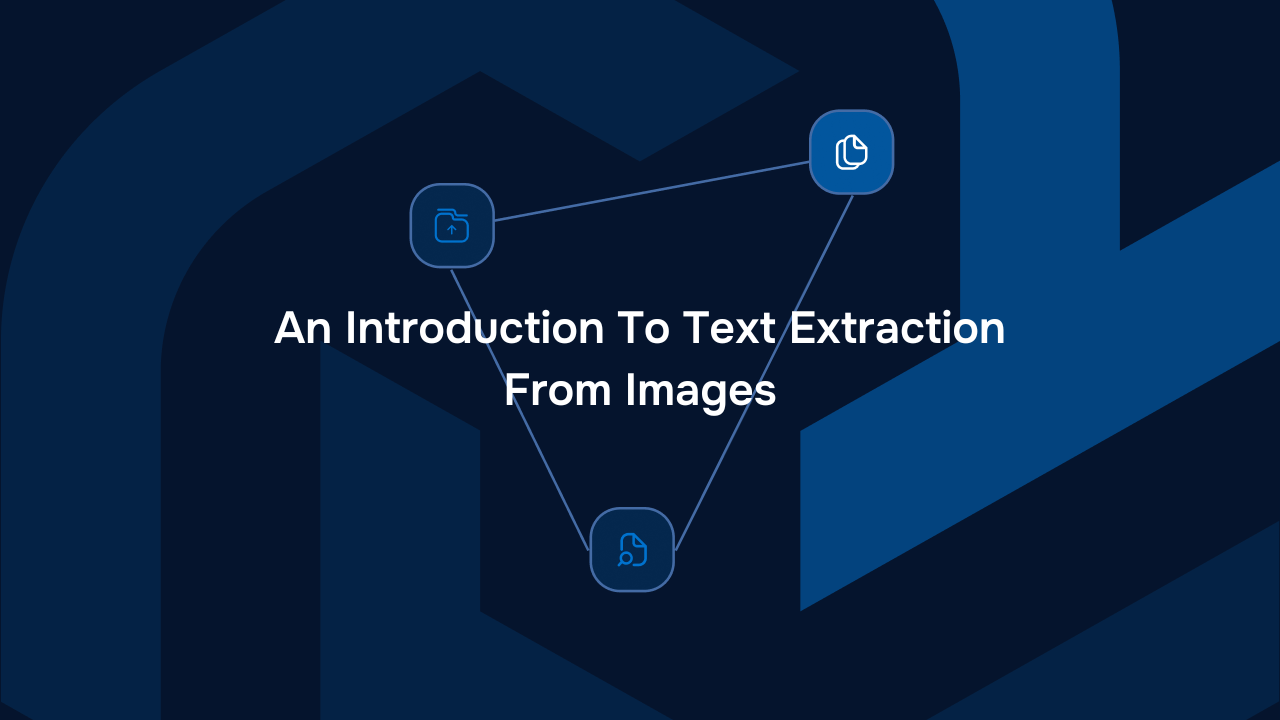
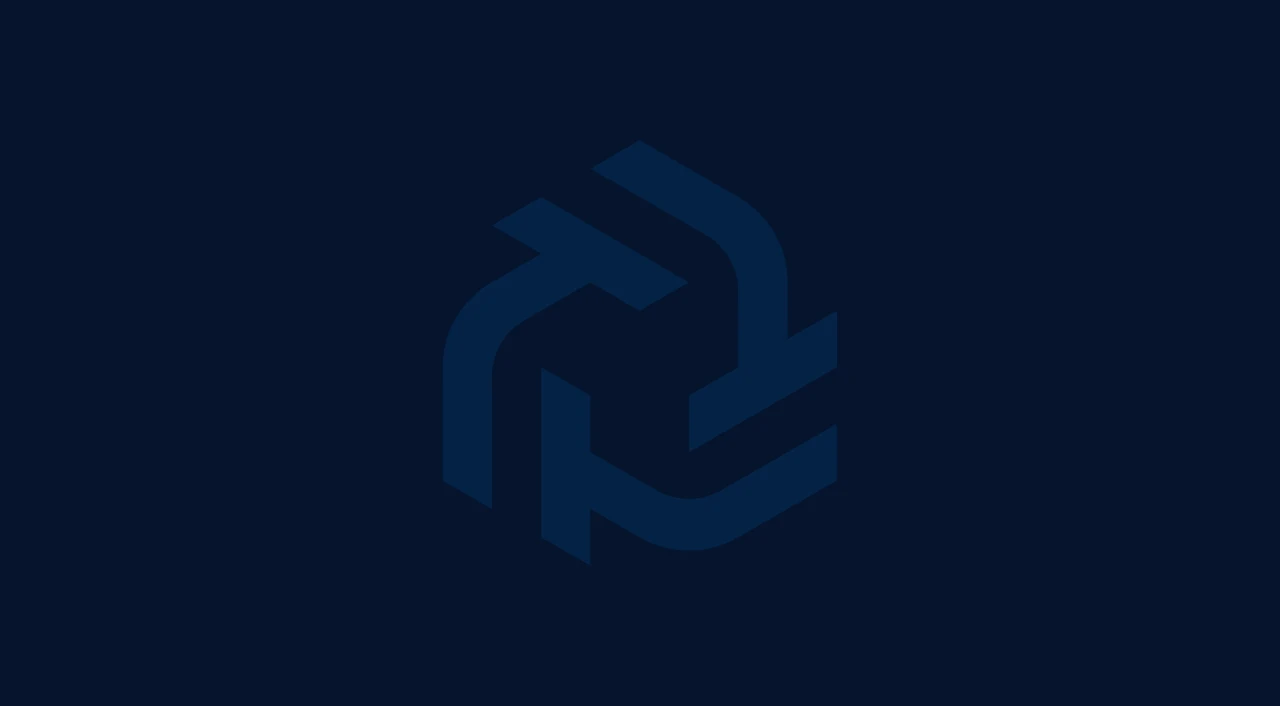
An Introduction To Text Extraction From Images
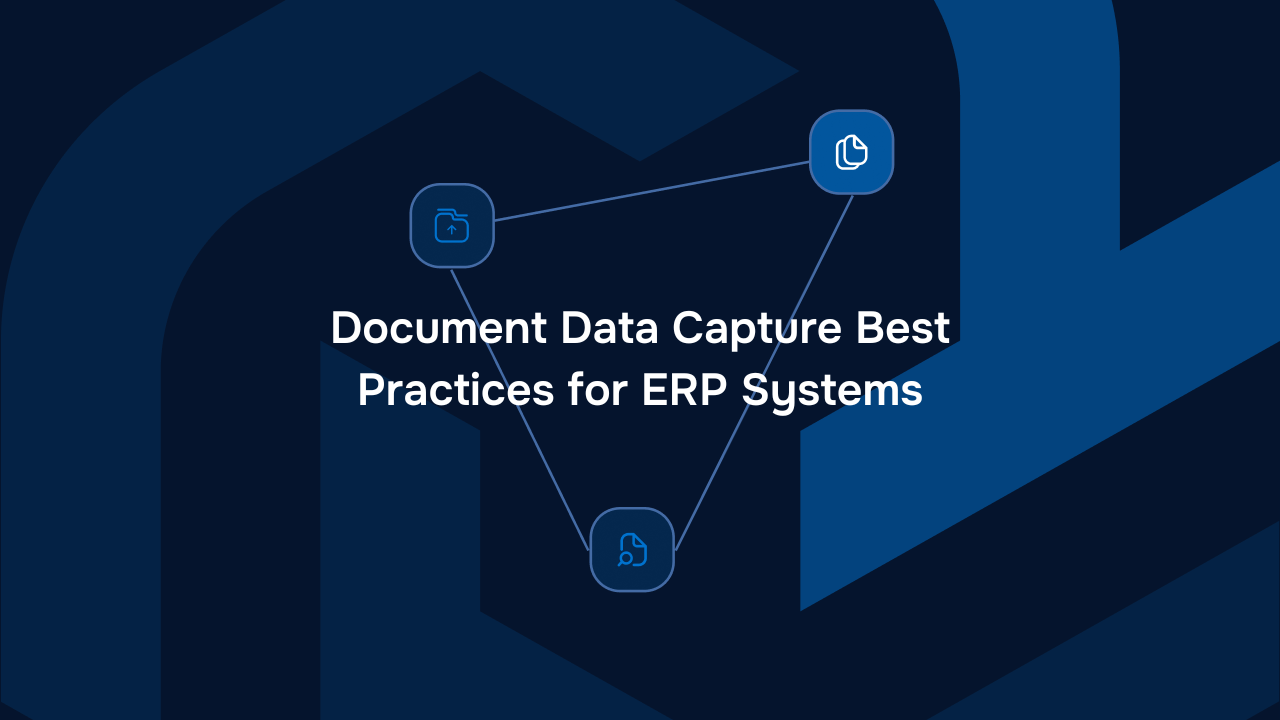
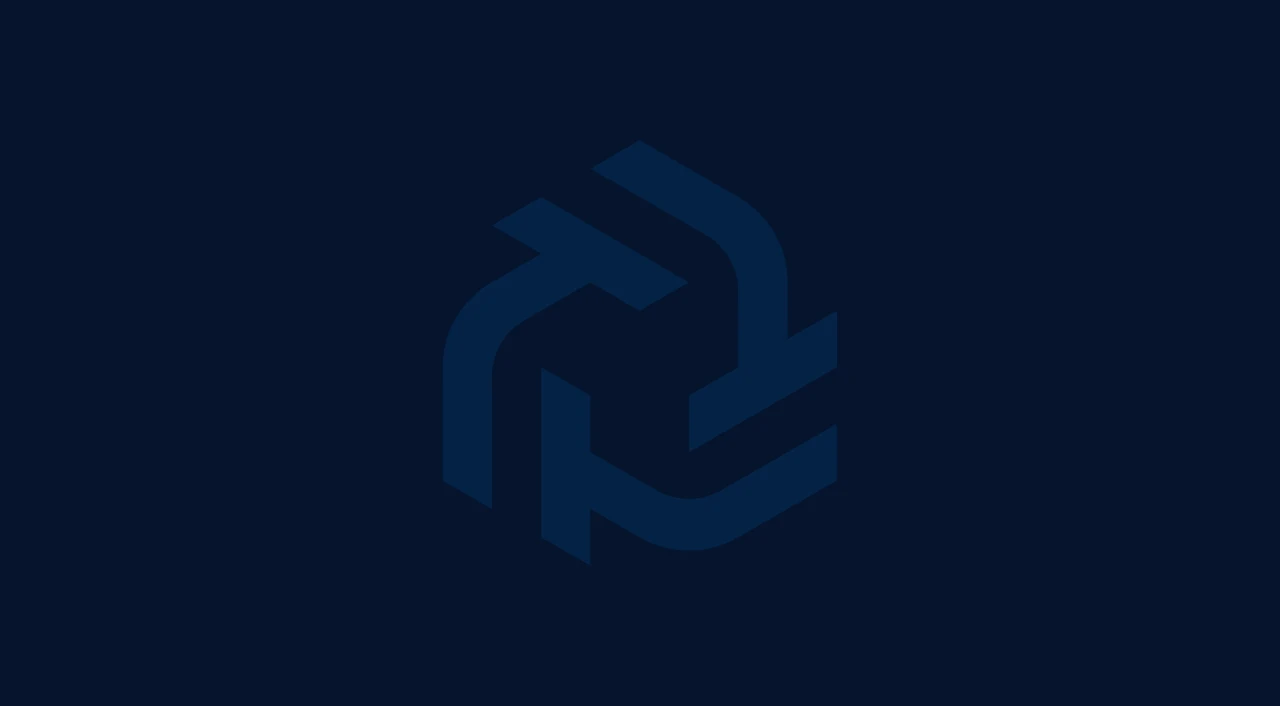