An Introduction to Generative AI
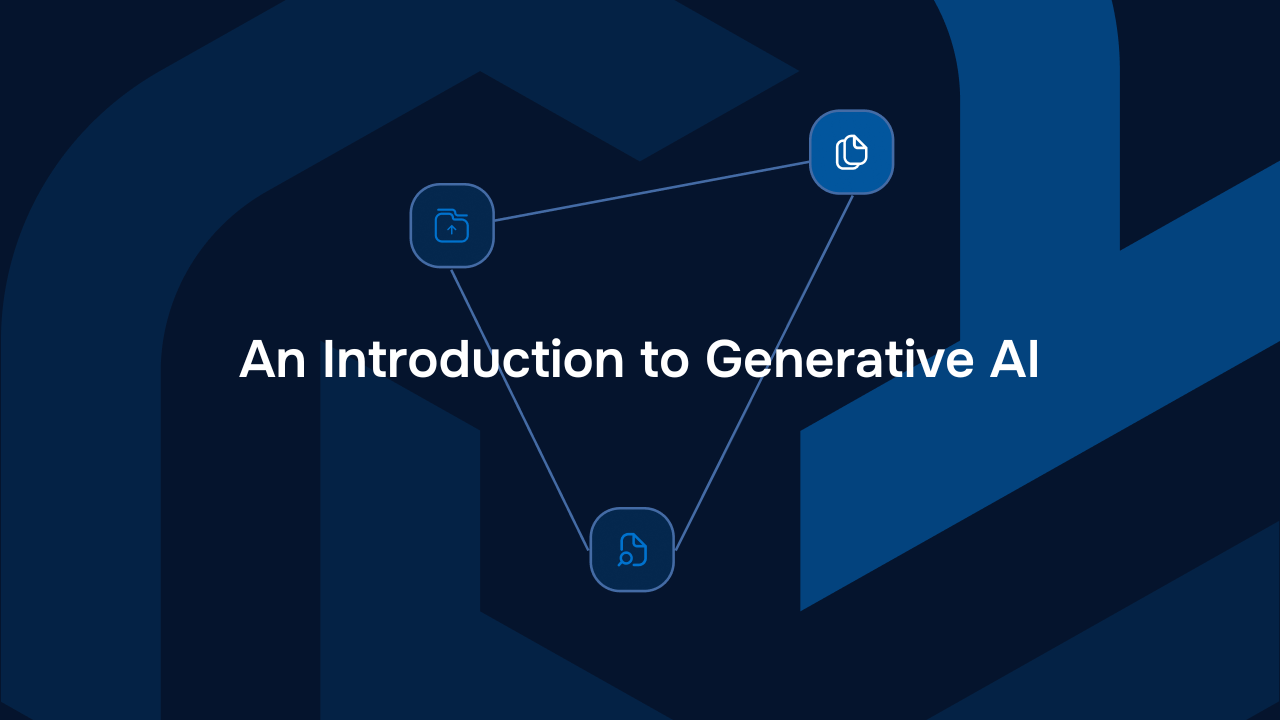
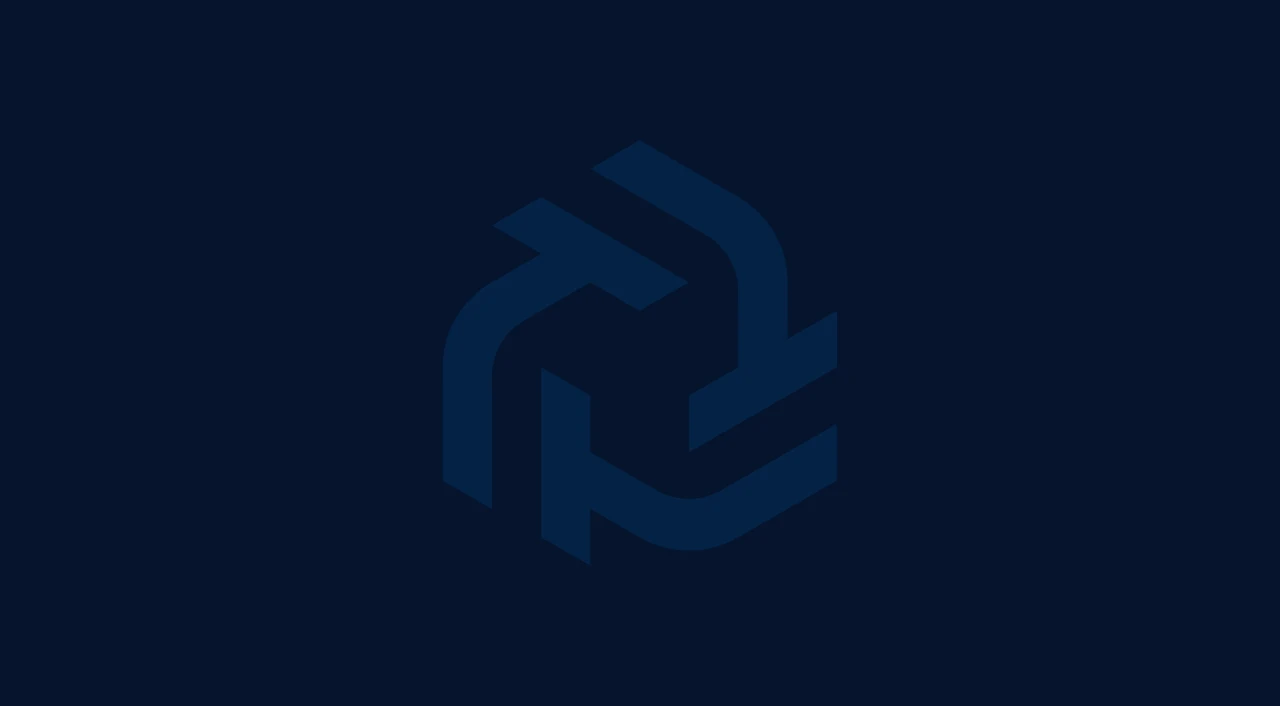
Machine learning algorithms are focused on identifying patterns in data whereas generative algorithms are a branch of artificial intelligence focused on generating data from prompts. Generative AI first took the world by storm in November 2022 when Chat GPT, a powerful chat-based agent was released to the public. Whilst text-based generative AI models, also known as large language models, are the most well-known, generative AI models can also generate audio, images and even video. This article provides an introduction to generative AI models and how businesses can harness their power to unlock efficiencies and unique insights.
What is Generative AI?
Generative AI describes any artificial intelligence model that generates content. This content can be text, images, audio or even video.
Rather than identifying relationships in existing content or predicting an output from input, generative AI algorithms attempt to generate an output which is illustrative of the input. The input of a generative AI model is often referred to as a prompt and provides instructions on the task of type of content it wants the model to generate.
For example, a prompt might ask the generative model to create an image of a person sifting through documents. Prompts can also include context which can be submitted for analysis. For example, a prompt might ask a large language model to summarise a paragraph or perform a text mining task.
Understanding Generative AI
Generative AI models are able to generate high-fidelity content because they are able to extract and make use of the underlying concepts of what it is they are generating. Generative models are able to uncover the underlying concepts without any form of supervision which is one reason why the models and applications are so versatile. However, the process by which the models extract the underlying concepts is not always explainable or correlated to how a human might go about extracting the core concepts.
For example, a generative model trained to generate images of humans will not know what a human hand is or how many fingers. Instead of learning these concepts and improving through knowledge, generative models have improved their performance primarily with more training data. New streams of research are looking at ontologies and knowledge graphs as a way of improving model performance more efficiently.
Types of Generative Models and How They Work
Generative models are built on the idea of combining a sampler with an autoencoder. An autoencoder is trained to capture the most important features of an input by encoding an input with a model and decoding the output of the encoder with another model. A sampler will feed new data points from the encoded space into the decoder in order to generate new content. The encoding and decoding is typically performed by neural networks and this type of generative model is referred to as a variational autoencoder (VAE).
Whereas VAEs are unsupervised, generative adversarial networks (GANs) are another type of generative model which frames the encoding and decoding as a supervised problem. As a result, a GAN will train two AI systems in parallel: a generating system, and a checking system also known as a discriminator.
The discriminator is trained on the original input and output dataset in order to classify whether the content generated by the generator is real or not. The generating neural network is trained until its performance outwits the discriminator. The generator can then be used as a generative model.
Autoregressive models are another key part of generative models as they are trained to predict the next element of a sequence. Large language models are built on the transformer architecture which is a type of autoencoder which uses the decoder for autoregressive natural language modelling.
What is next for generative AI?
Generative AI models have attracted a lot of attention with their ability to generate new content. However, the ability of a generative model to deconstruct underlying concepts is often more practical and valuable for businesses sitting on large quantities of unstructured data. This data can often provide context to the generative models in order to answer questions or solve specific tasks. For example, Vault leverages fine-tuned large language models to extract data from business-critical documents so that organisations can streamline a range of use cases including contract compliance and question answering.
How can businesses harness the power of generative AI?
In order to benefit from generative AI, businesses need to first understand the problems they have and whether it is suitable for a generative model. A problem which has lots of unstructured data or knowledge is most likely suitable for generative AI. For example, customer support agent response have improved significantly thanks to large language models. LLMs have also been a great way to make internal wikis more interactive because they are able to answer questions about them. Whilst organisations are already seeing the benefits of generative AI and initiating training to encourage adoption, generative AI is still in its infancy both in terms of functionality and use cases. Generative AI will increase productivity and efficiencies so dramatically over the next couple of years that organisations which don’t use it will be left behind.
About TextMine
TextMine is an easy-to-use data extraction tool for procurement, operations, and finance teams. TextMine encompasses 3 components: Legislate, Vault and Scribe. We’re on a mission to empower organisations to effortlessly extract data, manage version controls, and ensure consistency access across all departments. With our AI-driven platform, teams can effortlessly locate documents, collaborate seamlessly across departments, making the most of their business data.
Newsletter
Blog
Read more articles from the TextMine blog
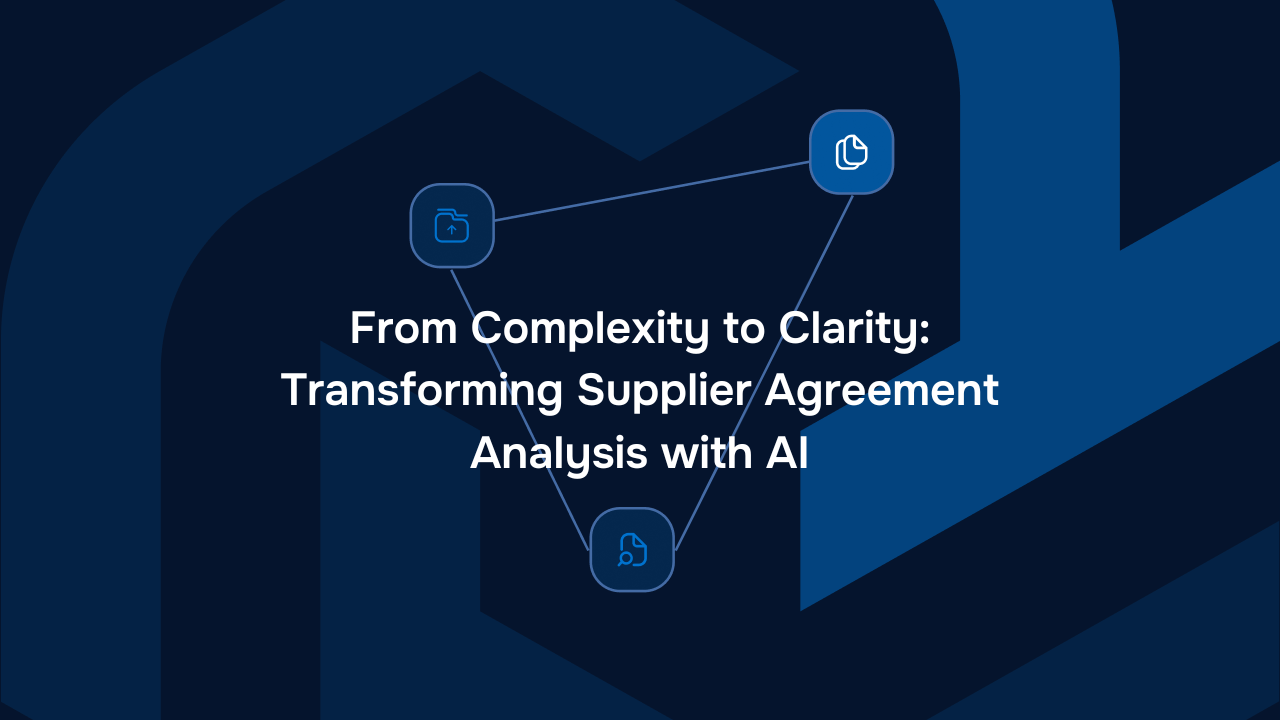
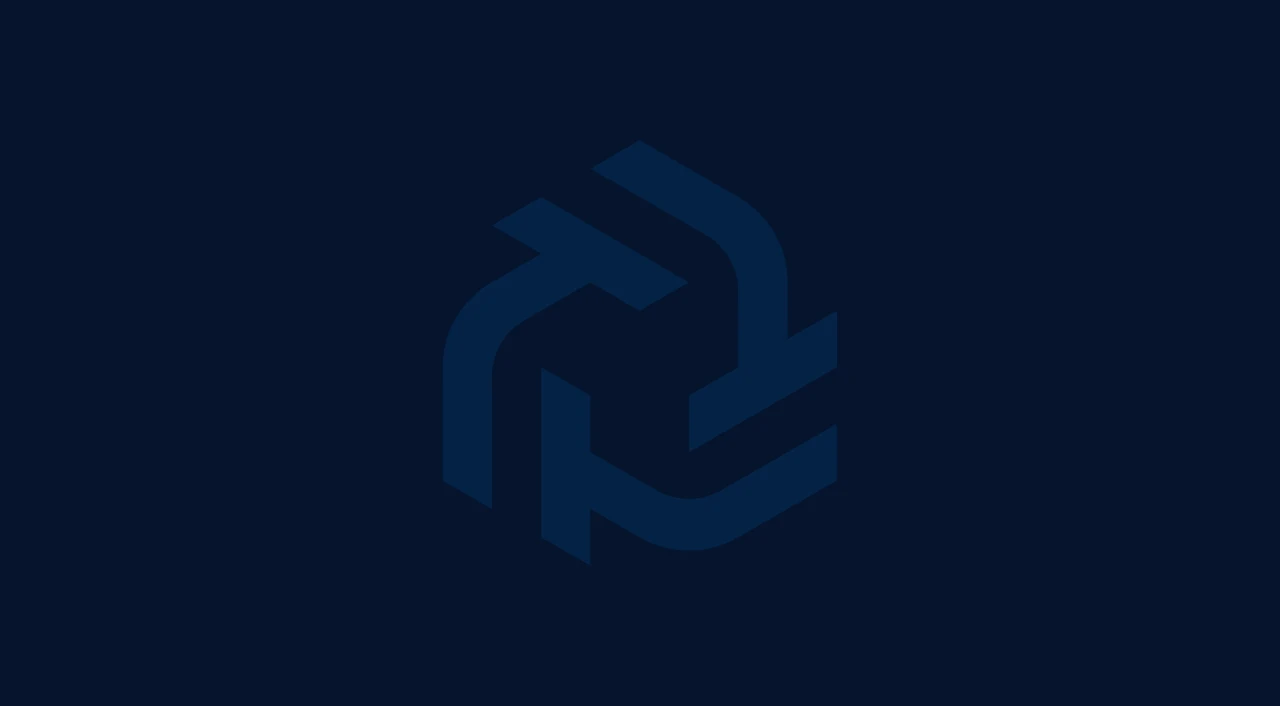
From Complexity to Clarity: Transforming Supplier Agreement Analysis with AI
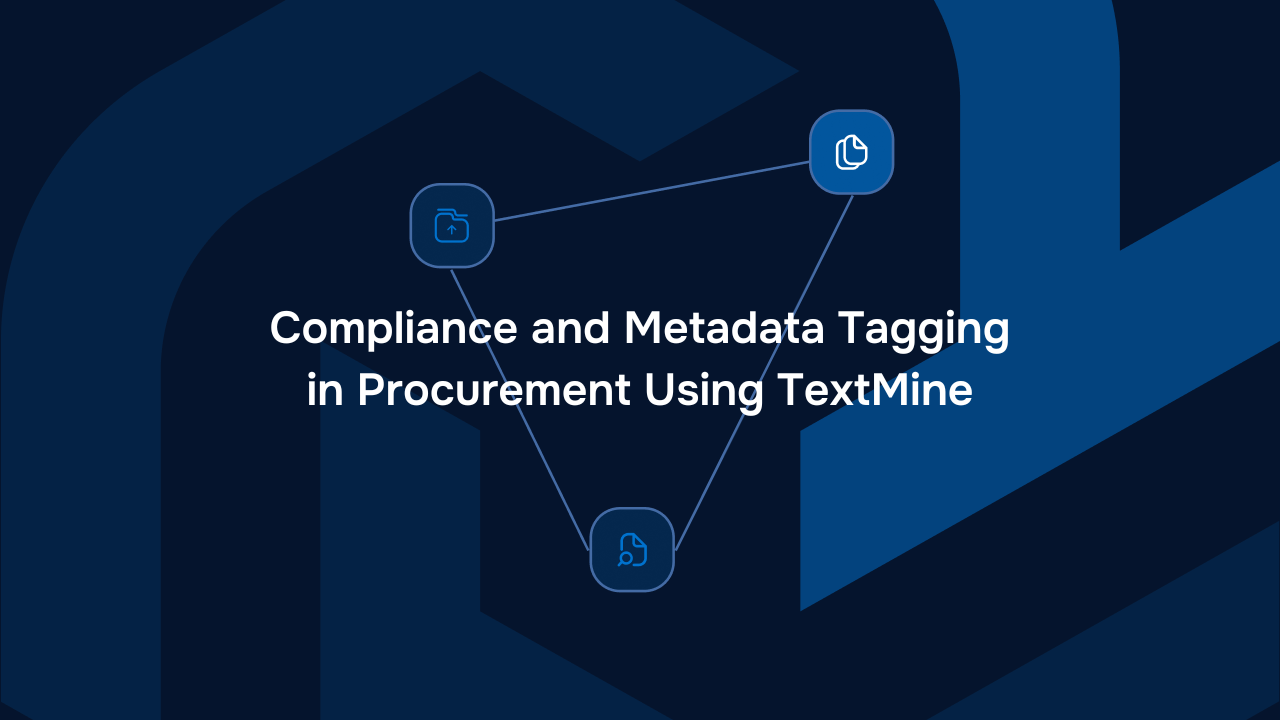
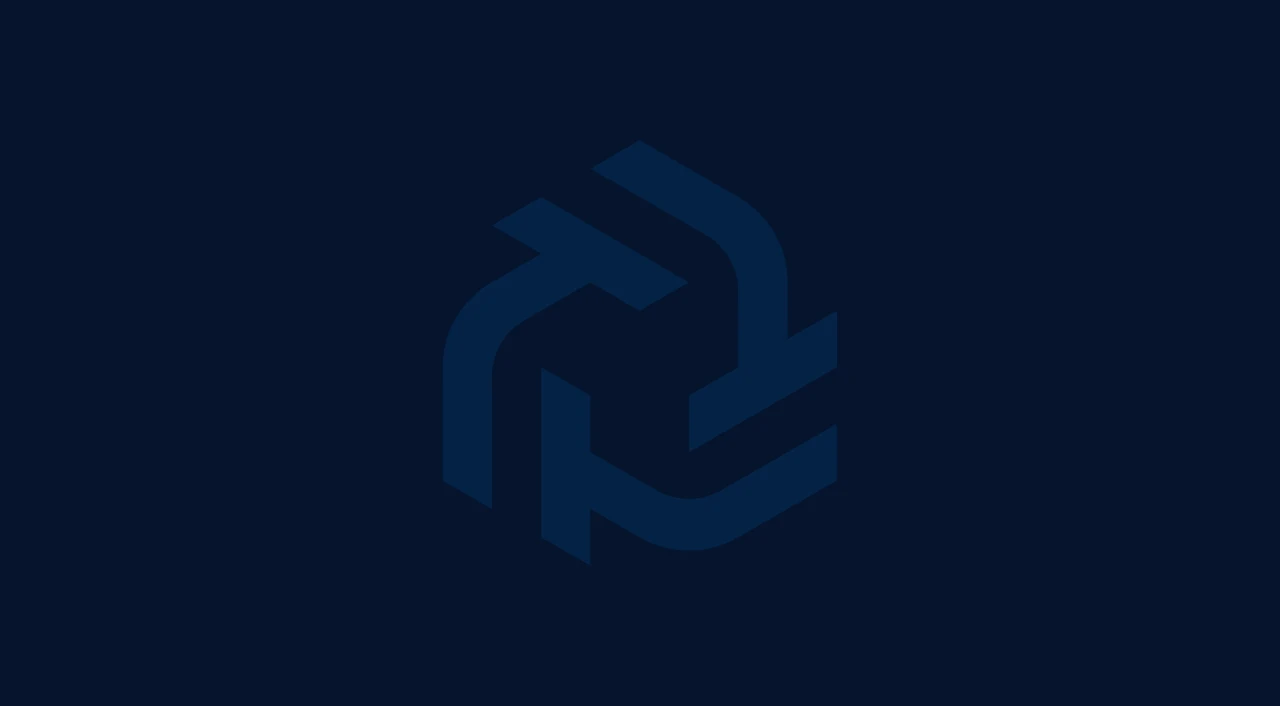
Compliance and Metadata Tagging in Procurement Using TextMine
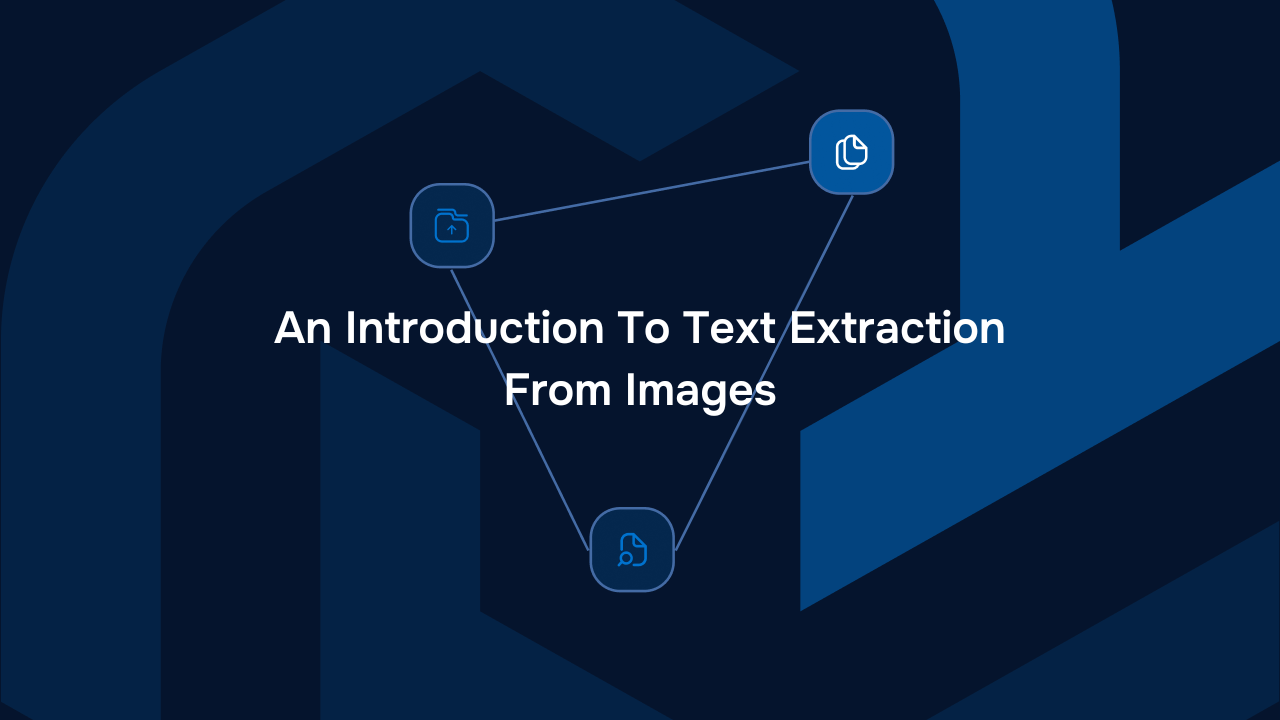
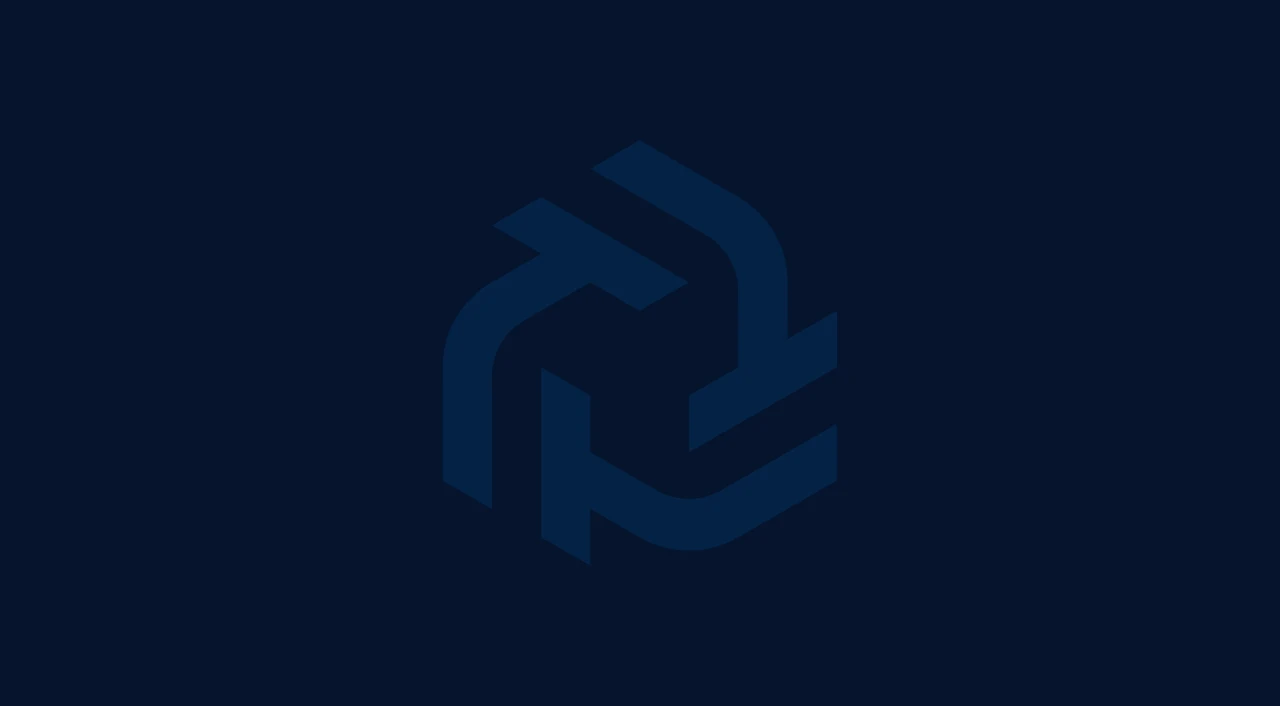
An Introduction To Text Extraction From Images
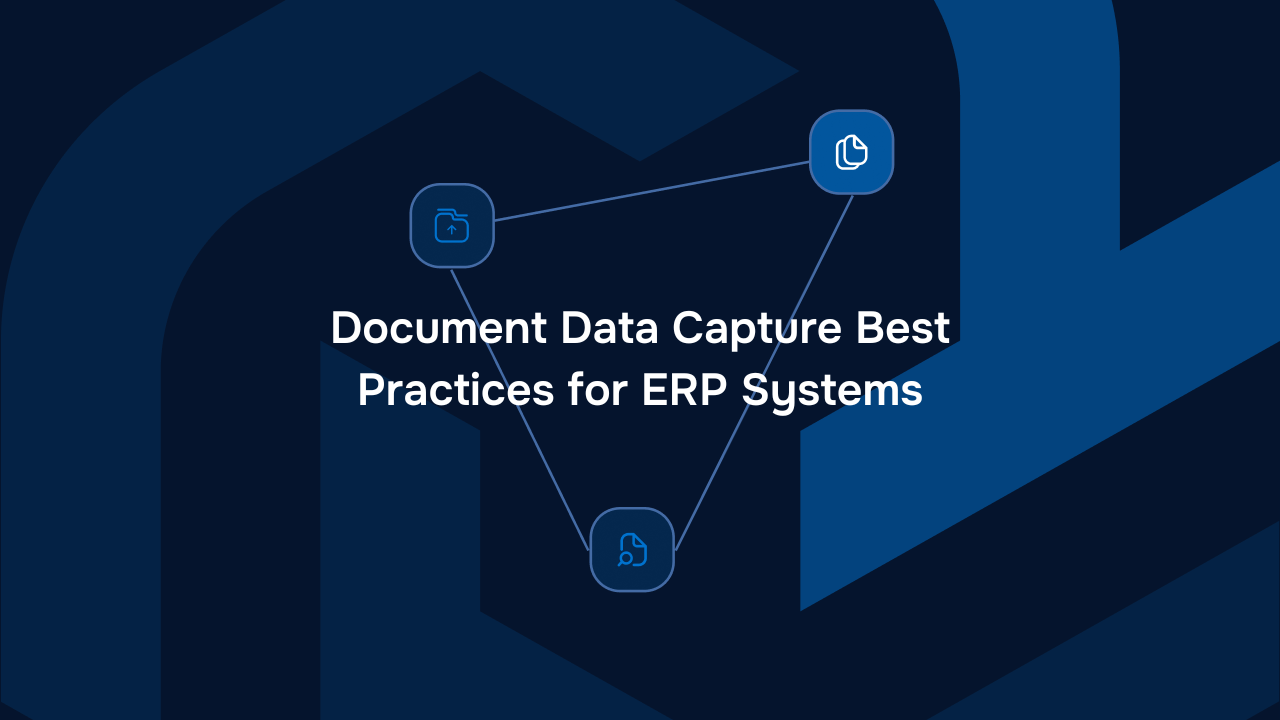
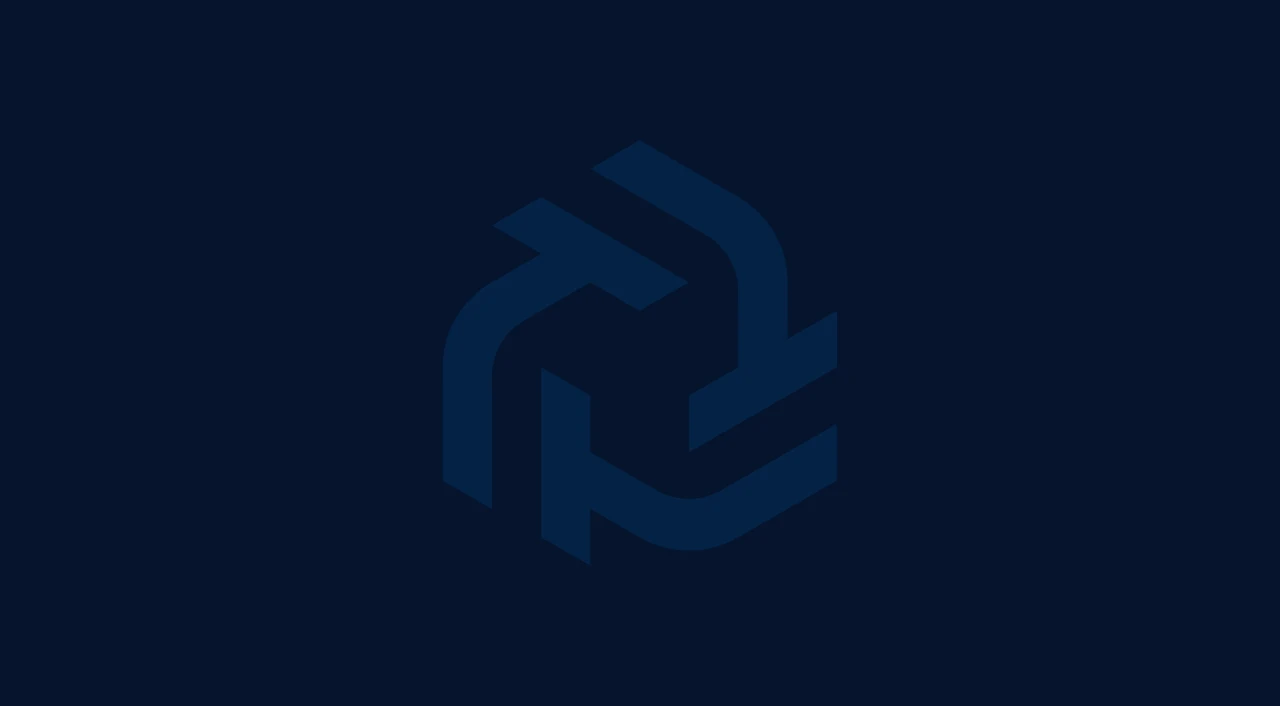