How to Manage the Risks of AI
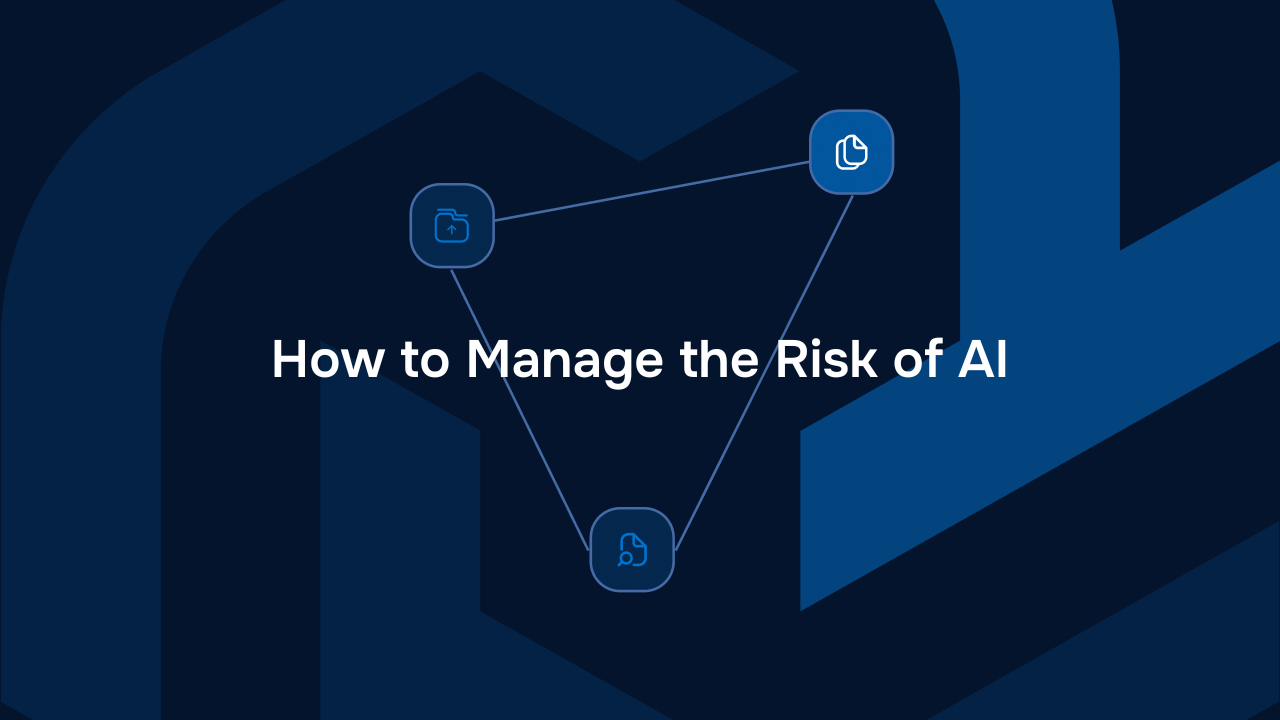
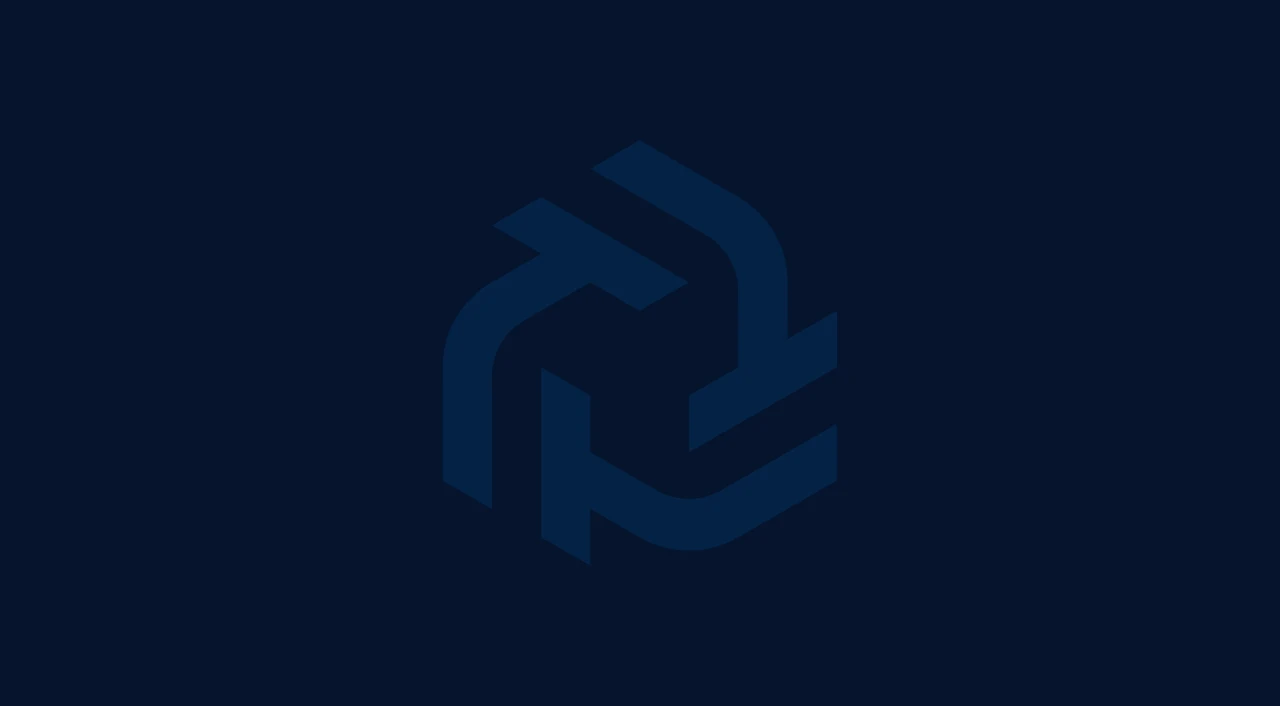
One of the biggest obstacles to AI challenge is the fear of the risks of AI. AI can radically transform an organisation by unlocking efficiencies and new insights. However, without a proper risk management framework, an AI model implementation can present a number of flaws such as ethical issues and biases. This article provides a framework to help organisations better mitigate the risks of AI in their digital transformation projects.
The Rise of AI
Over the years, AI has become not only far more advanced but has also seen a huge spike in popularity with the popularisation of generative AI tools like Chat GPT. As a result, AI has gone mainstream and more and more organisations are looking at how to implement AI into their business operations. Unfortunately, while many people are aware of the existence and convenience of AI, they are not always as aware of the potential risks.
The Potential Risks Involved With AI
Before introducing AI into your organisation, there are a range of factors that you may want to look out for when it comes to AI usage.
Bias and fairness
AI models are trained using data which means that they may present biases if the underlying data they have been trained on contains bias. For example, if an AI is trained to detect swans and only sees images of white swans, it will be biassed if it is not exposed to training data which includes southwest Australian swans which have black feathers. If the data that the AI model has been trained on contains any biassed, unfair, or discriminatory information, this can be reflected in the content that the AI model produces. As a result, it is important to review the training data to determine if it has these fundamental flaws before training any type of model. As a solution, the training data can be corrected and expanded to compensate for these biases and make the model fair.
Security concerns
Machine learning and AI models are fine-tuned to a data set which means that their parameters are connected to the underlying data. As a result, an AI tool will often collect and store data and information. Some security concerns can arise if this this data is sensitive and the AI model or service provider does not implement adequate security and privacy controls.
Lack of explainability
AI models which are not linear such as generative and deep learning models are not natively explainable. As a result, they can’t be used in high stakes or regulated environments where mistakes are costly or predictions need to be explained. Traditional linear based machine learning models are natively explainable and can therefore be a good option if your use case requires explainability. Another option for providing explainability to generative and deep learning models is to do analysis of the inputs with the outputs and involve a human in the loop to monitor the model’s behaviour.
Ethical and privacy issues
As AI models become more powerful and learn to automate tasks, there are some concerns that they will take jobs from real humans in fields such as visual art, writing, coding, and music production. There are also some concerns with the amount of data that AI models collect, as well as the source of this data, especially if it has been scraped from websites. There is a risk of AI models scraping data from those who have not consented to their work being used, such as independent online publishing platforms and visual art posted to social media. However, whilst AI will automate tedious jobs, this will free up time for more creative and higher value tasks.
Unintended consequences
Overall, there are a range of different unintended consequences that can stem from the use of AI. Job displacement, security issues, unethical data scraping, and bias or discrimination are just a few of the consequences that we have seen stem from AI use. AI innovation is also evolving rapidly which is why it’s important to keep up-to-date with AI risks and regulation.
How to mitigate the risks of AI
There are a few key steps that organisations can take to mitigate AI risks:
- Regular risk assessments – Implement regular risk assessments and ensure that all of the data that the AI model is being trained on, as well as the output, is correct and ethical
- Follow ethical guidelines – Confirm that the AI model or bot that you are training or working with is following all relevant ethical guidelines in terms of data acquisition
- Have robust data privacy measures – Put strict data privacy measures in place and ensure that they are being followed in order to avoid any data leaks or privacy breaches
- Continuous monitoring – Continue to closely monitor the AI to ensure nothing goes awry and the content remains accurate and unbiased
- Transparency in AI systems – Having a transparent AI system is a great way to make sure users understand how the model functions
- Bias detection and mitigation – Implementing bias detection is one great way to ensure the AI is not producing discriminatory or inaccurate content
- Clear accountability – Maintaining clear accountability is crucial when it comes to ensuring an AI model is well respected and trusted
- Compliance with regulations – Something that is vital when it comes to the use of AI is making sure that the model or bot is compliant with any and all applicable regulations
- Adequate cybersecurity measures – Having good cybersecurity measures in place when working with an AI model is going to help protect private data, eliminating security issues further down the line
- Regular updates and maintenance – AI models will often require regular updates and maintenance in order to run smoothly. This is something that can improve both the AI interface as well as the content it produces.
Conclusion
AI is a powerful technology that can be of great use to organisations in many different fields. That being said, there are also a wide range of risks that can come along with the implementation of AI. However, by understanding the risks and monitoring them, organisations are better equipped to mitigate the potential risks of AI.
About TextMine
TextMine is an easy-to-use data extraction tool for procurement, operations, and finance teams. TextMine encompasses 3 components: Legislate, Vault and Scribe. We’re on a mission to empower organisations to effortlessly extract data, manage version controls, and ensure consistency access across all departments. With our AI-driven platform, teams can effortlessly locate documents, collaborate seamlessly across departments, making the most of their business data.
Newsletter
Blog
Read more articles from the TextMine blog
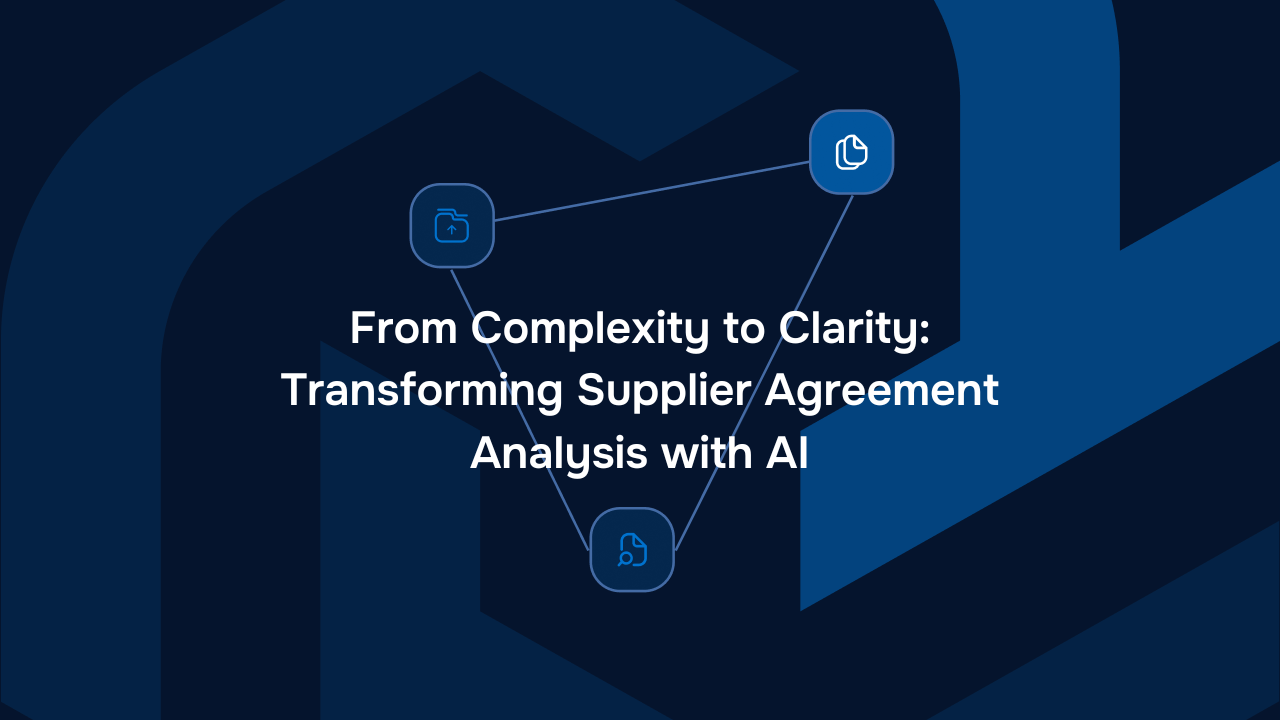
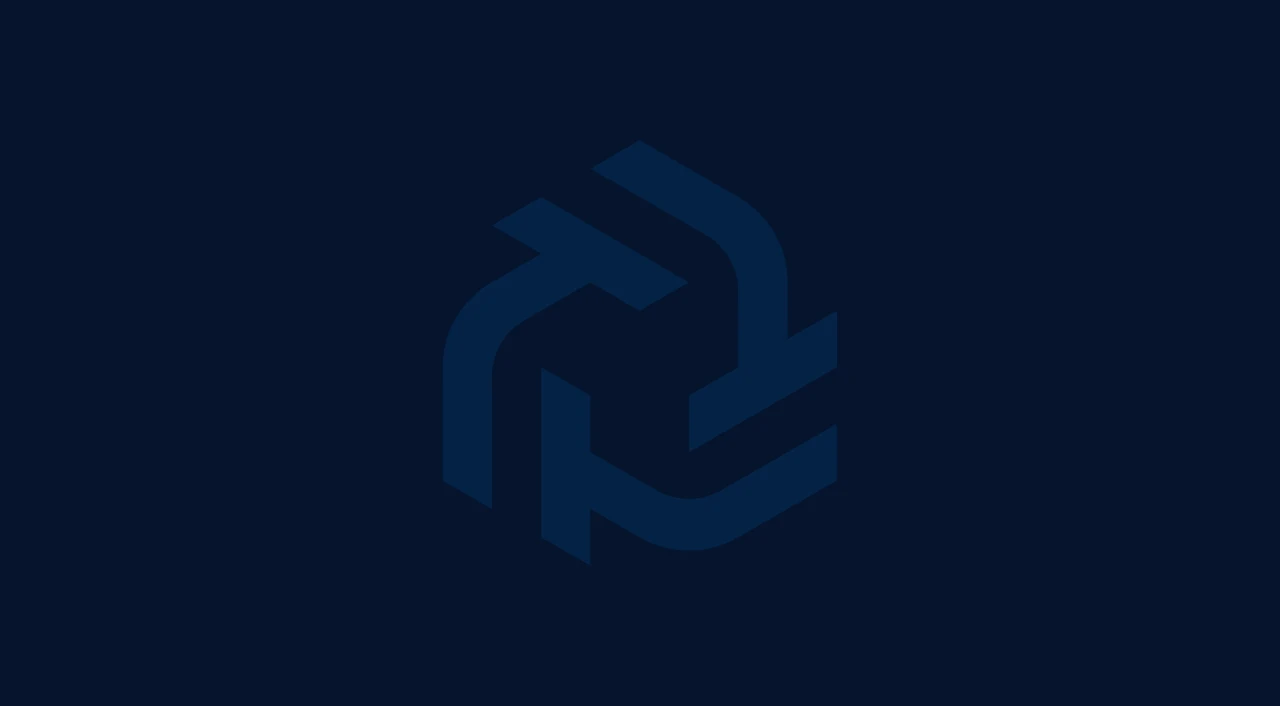
From Complexity to Clarity: Transforming Supplier Agreement Analysis with AI
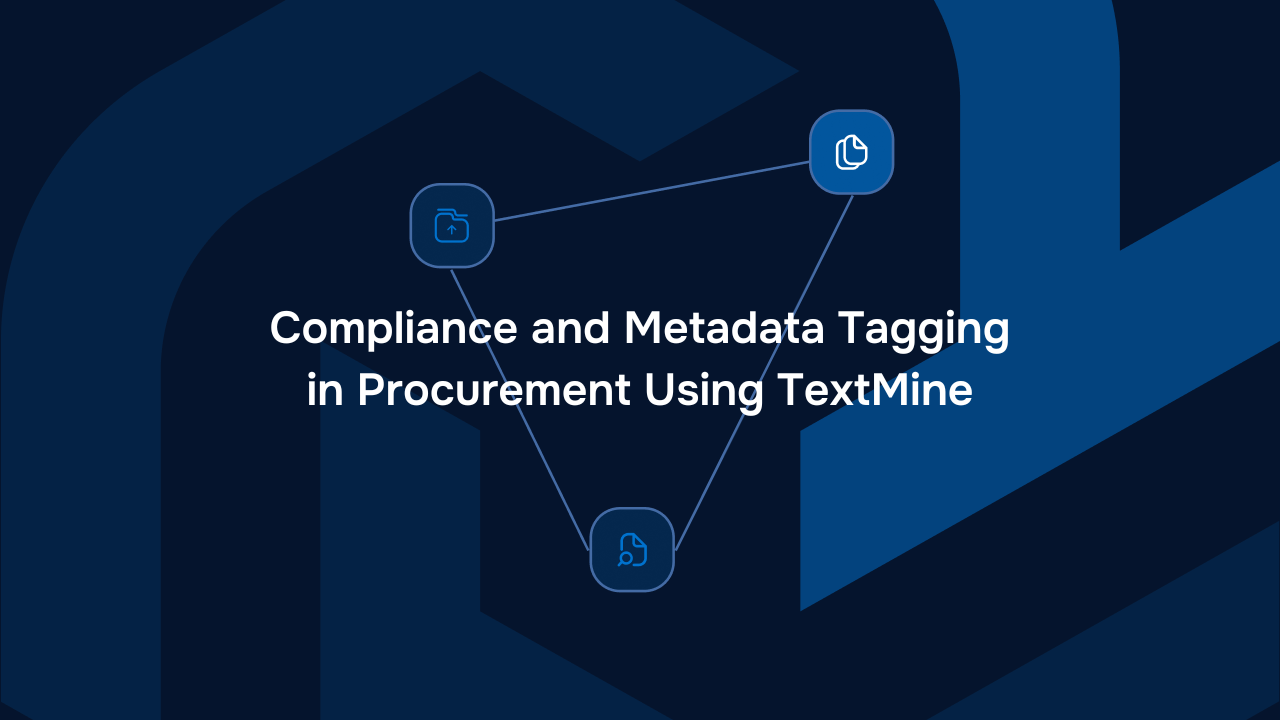
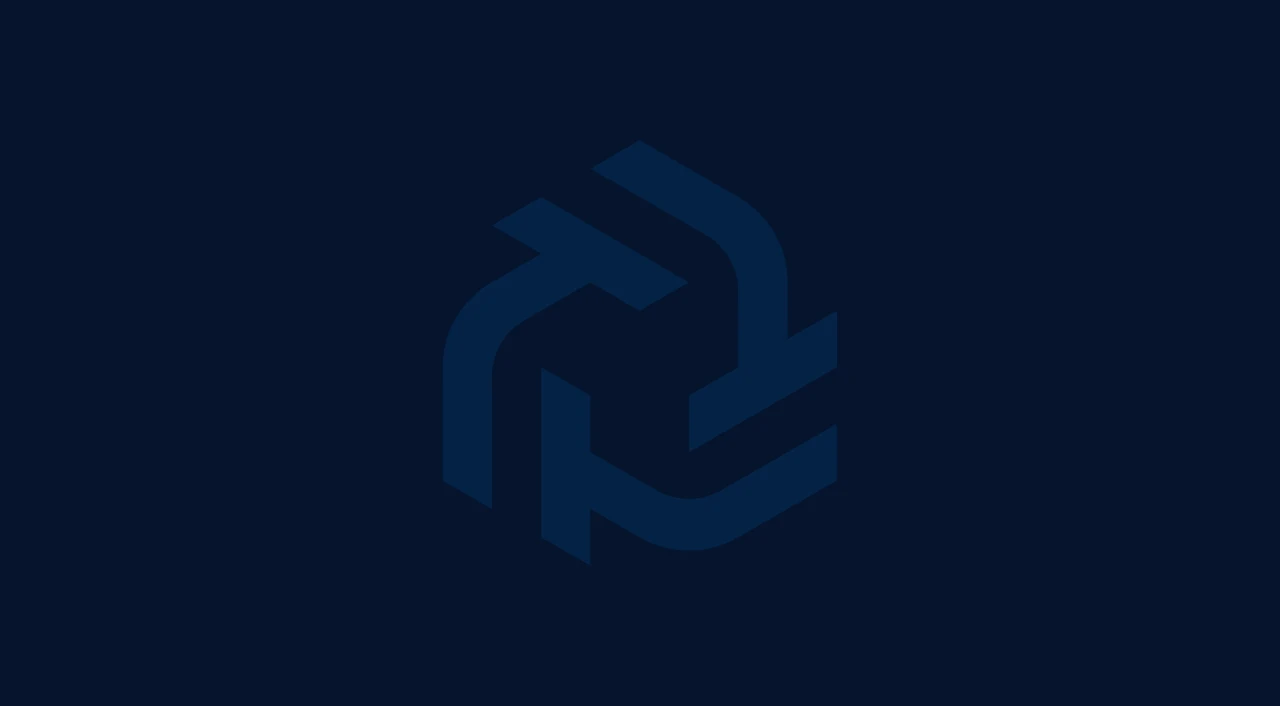
Compliance and Metadata Tagging in Procurement Using TextMine
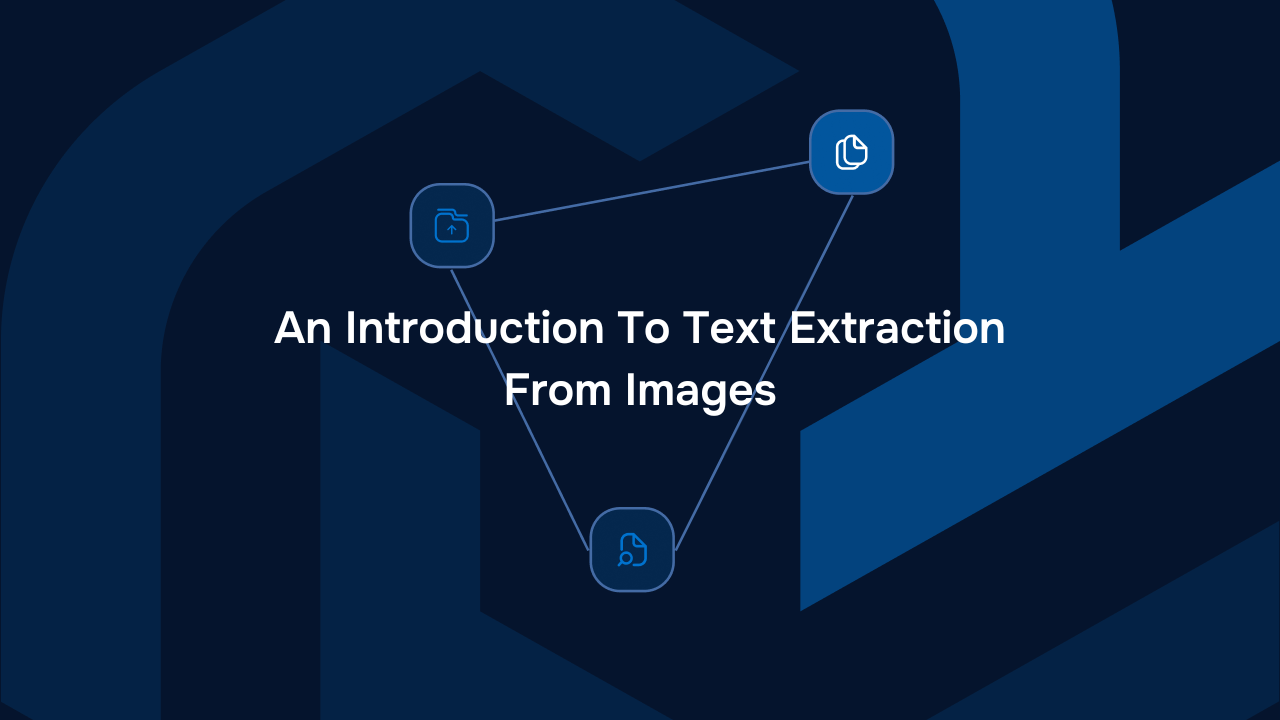
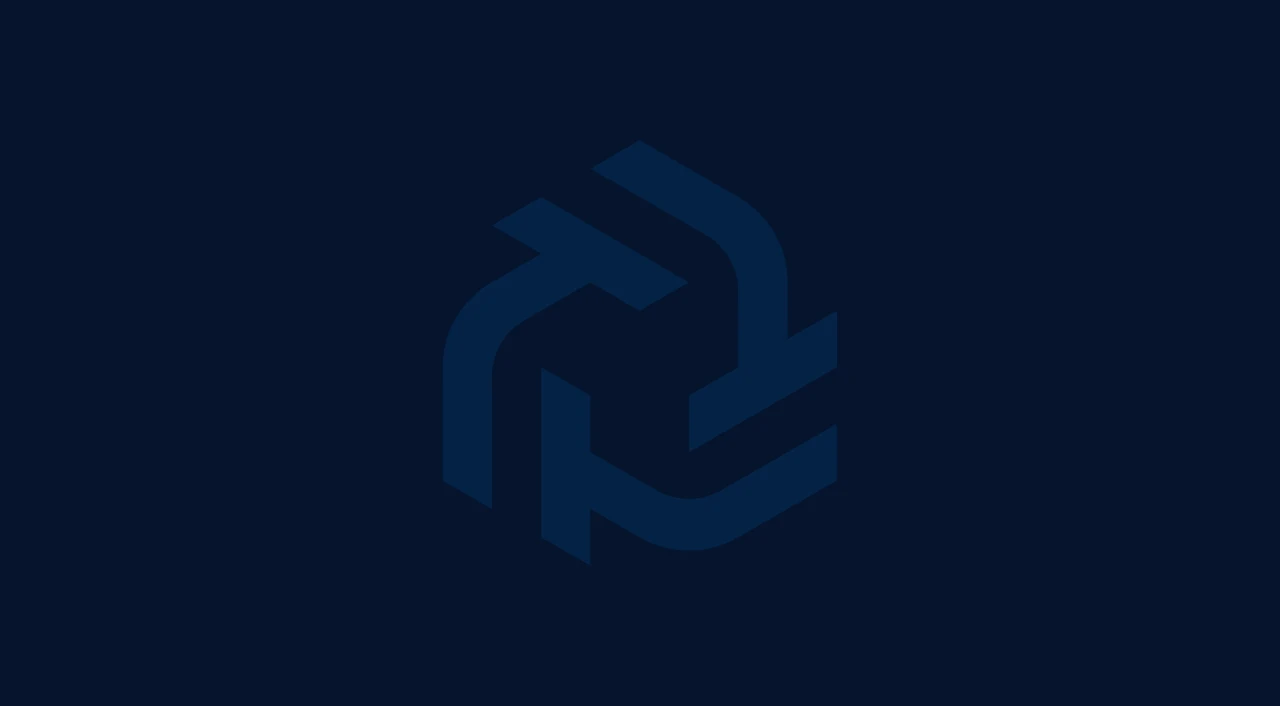
An Introduction To Text Extraction From Images
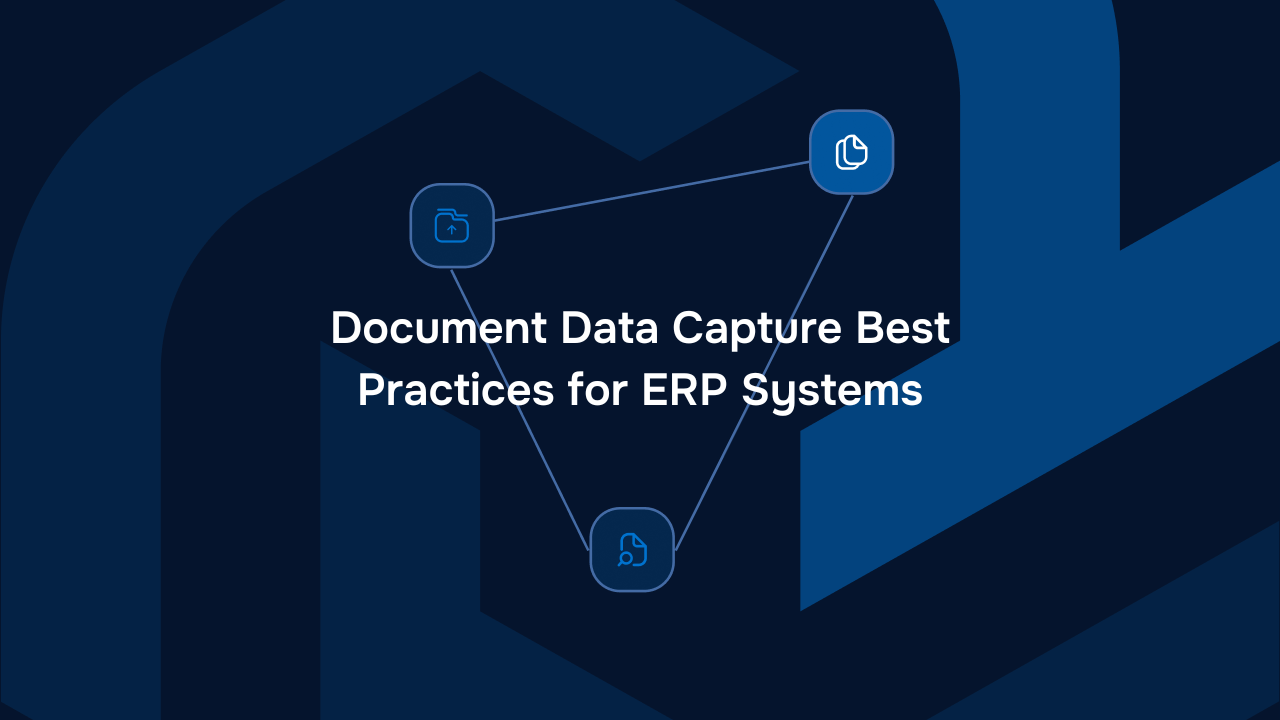
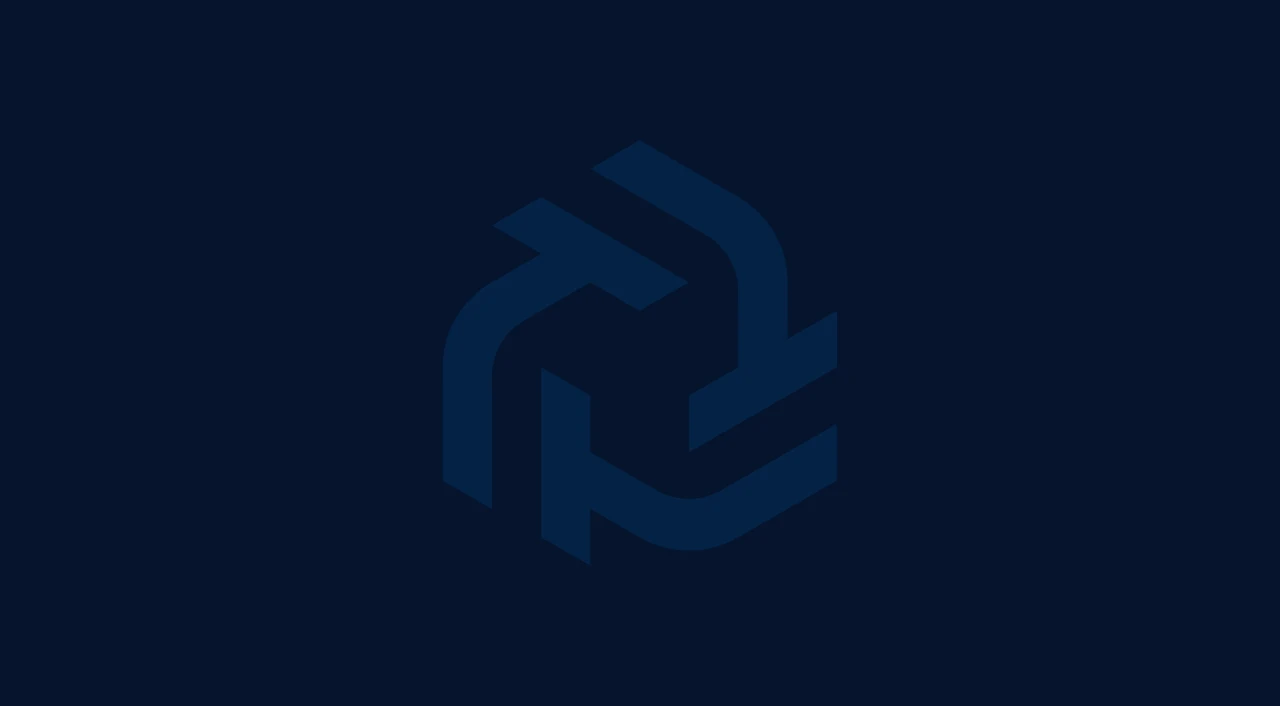