Text Analytics Vs Text Mining
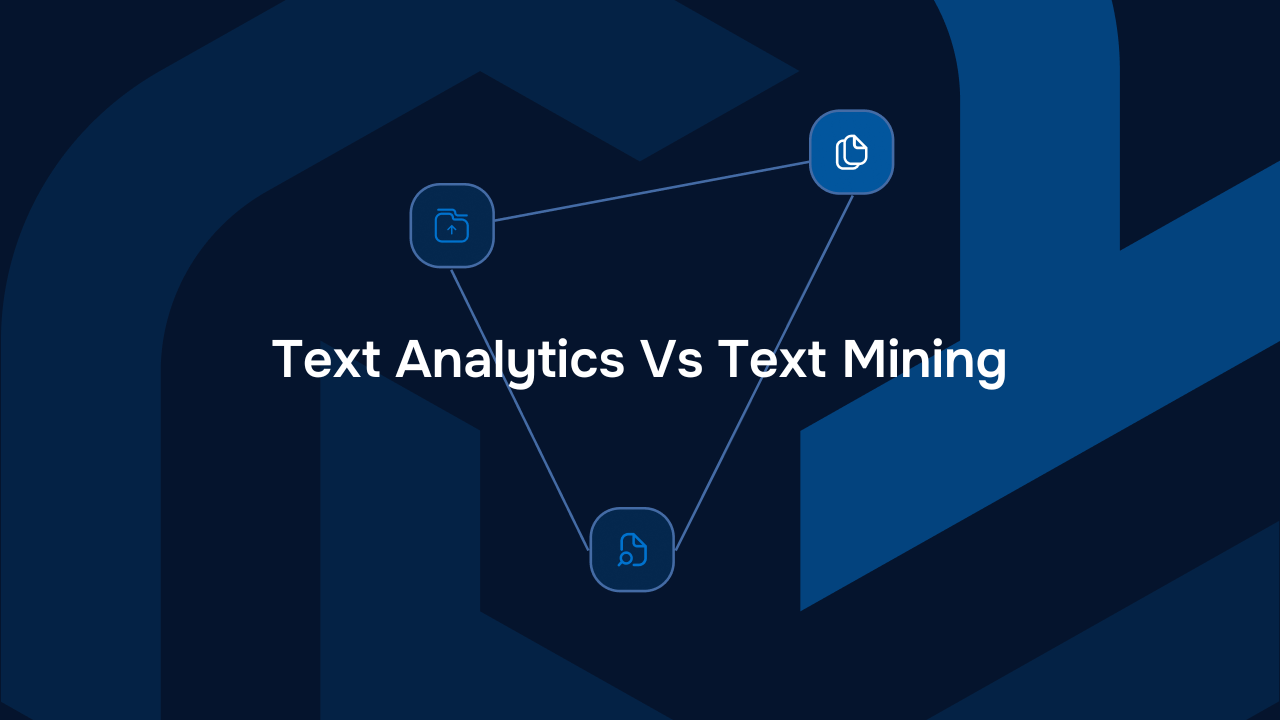
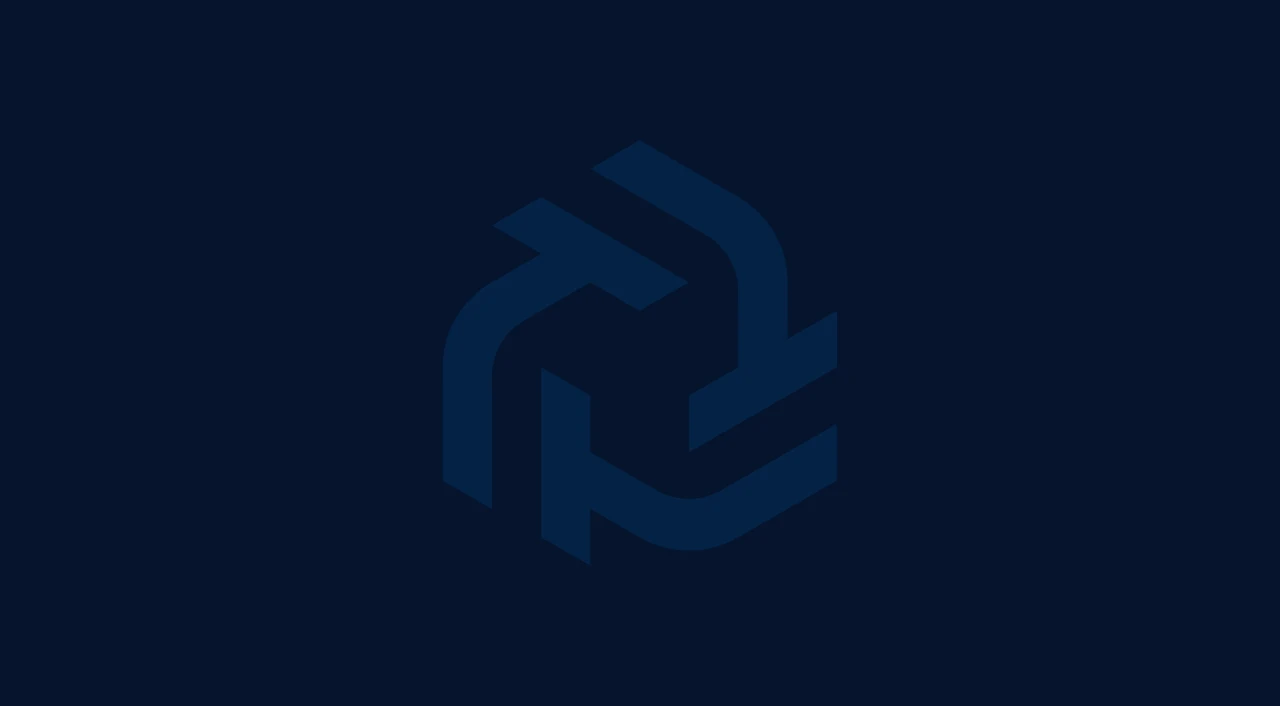
In today’s business landscape, data is king. Collating, interpreting, and gaining insights from data is necessary to ensure your business is running efficiently and making data-driven decisions..
Text analytics and text mining are both used to gain insights from text, and though similar they have key differences. This article breaks down the two techniques, helping you understand how they work and their key applications. We cover:
- What text analytics is
- What text mining is
- The main differences between the two techniques
- Business applications of the techniques.
What is Text Analytics?
Text analytics is a process that breaks down text into its properties for further analysis. This data can then be analysed by using algorithms and natural language processing techniques, which can find out patterns and trends.
How it works
Text analytics works by breaking up longer sentences in the overall text into their components, and then using analysis to discover insights.
The text can be sourced from a variety of places such as emails, customer reviews, or social media posts. Before analysis, the text is cleaned and normalised and can then be analysed to find out trends and patterns.
What is Text Mining?
Text mining refers to the process of extracting valuable information from text. Like text analytics, it uses various techniques to process unstructured text and find patterns.
How it works
The text mining process begins with the collection of raw textual data. Like with text analytics, this data can be gathered from a variety of places depending on your goals, including social media, emails, online posts or even contracts!
Text mining identifies relevant information with a collection of text, giving qualitative results that can help businesses make decisions.
Text Analytics vs Text Mining: Their Main Differences
Although both text mining and text analytics are used to analyse raw textual data, they use different techniques and provide different types of results. In short - text mining is looking to infer insights from the intrinsic text while text analytics is look to describe the descriptive properties of text.
Focus on analysis
Text analytics is focused on getting quantitative data from tests, and then analysing that data using statistics to find patterns and trends.
Text mining, on the other hand, aims to find hidden insights, unexpected relationships, and structures between elements in the text. Text mining leverages NLP, machine learning and generative AI to uncover patterns from text based data.
Application areas
Text analytics is useful in areas such as customer service and social media monitoring.
It’s a great way to gauge trends in sentiment and categorise different types of feedback!
Text mining is often used in research and development and looking for specific information. It has applications in fraud detection as well as contract audits.
Output
The output of text analytics is usually in the form of reports, structured data, and clear insights. These results can be used to inform business strategy and decision-making.
Conversely, text mining can lead to the discovery of brand-new concepts and ideas, which makes it more valuable for investigative research and exploring new facets.
Use Cases in Business Applications
Both text analytics and text mining are valuable tools across many business sectors. Both can be used to your advantage to improve your operations and long-term growth and planning - let’s break down their main applications.
Text Analytics
- Customer feedback analysis: Many businesses use text analytics to analyse the feedback they get from customer surveys, reviews, and feedback forms.
This is a great way to discover trends in and respond to common issues, get an idea of overall satisfaction levels, and find out how to improve customer experience.
- Social media monitoring: Social media insights are great for monitoring brand perception trends and customer sentiment. It also allows responding to trends in real-time, which improves customer engagement.
Text mining
- Fraud detection: Text mining techniques can detect fraudulent activities by discovering irregularities in text in emails, transactions, and other types of data. This helps reduce fraud risk and protects businesses.
- Competitive intelligence: Text mining can also give businesses a competitive advantage by discovering trends and unexpected moves being made by a competitor.
- Contract management: Text mining can be used to track contracts throughout their lifecycle. This is more efficient than using a shared drive and spreadsheets for tracking contracts and other business-critical documents.
Conclusion
While both text mining and text analytics are strategies for parsing the data from unstructured text, they offer different benefits! Text analytics is essential for a quantitative analysis of trends, and descriptive properties of text. Text mining can be used for finding unexpected patterns which occur within your text, which can be particularly helpful for uncovering fraud and tracking your legal and financial obligations in contracts.
About TextMine
TextMine is an easy-to-use data extraction tool for procurement, operations, and finance teams. TextMine encompasses 3 components: Legislate, Vault and Scribe. We’re on a mission to empower organisations to effortlessly extract data, manage version controls, and ensure consistency access across all departments. With our AI-driven platform, teams can effortlessly locate documents, collaborate seamlessly across departments, making the most of their business data.
Newsletter
Blog
Read more articles from the TextMine blog
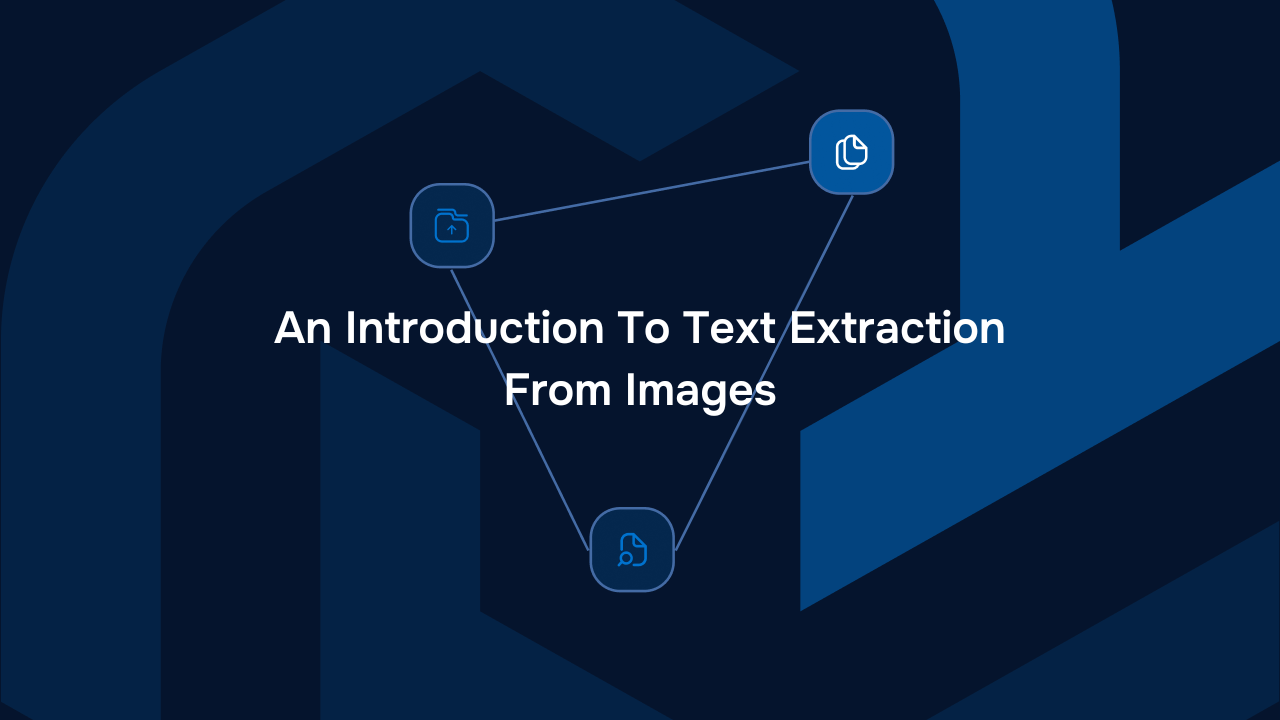
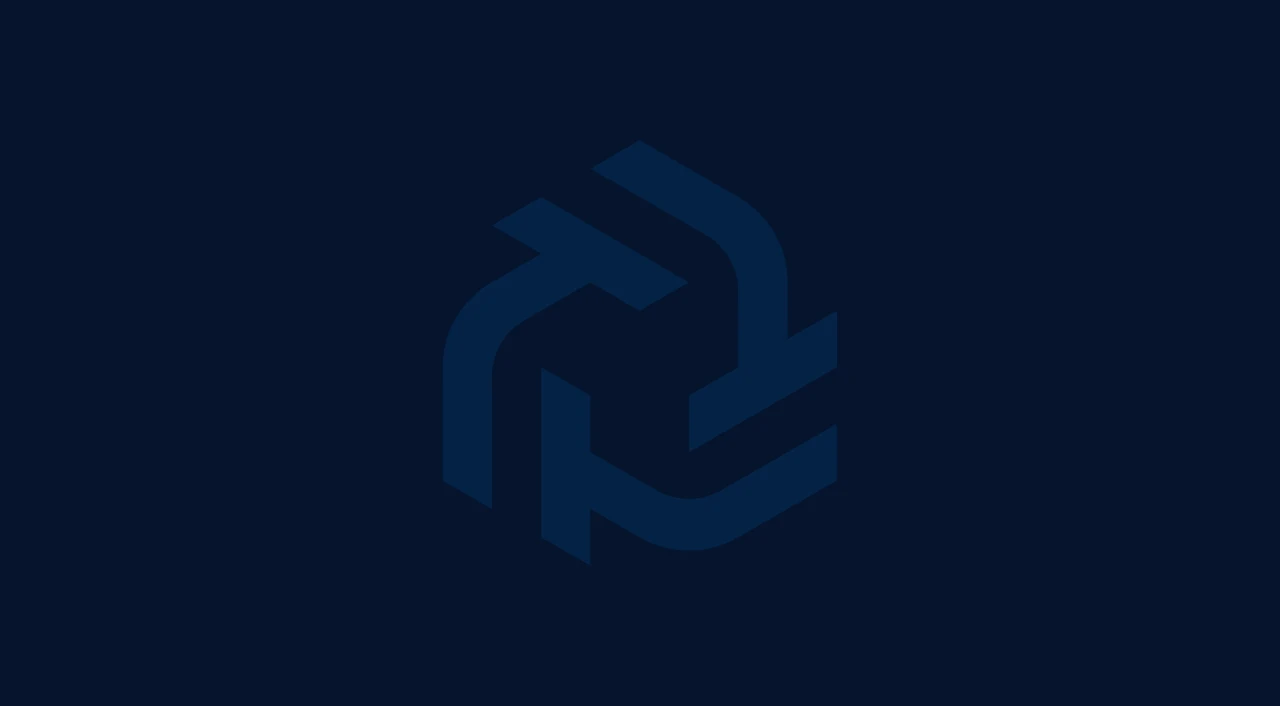
An Introduction To Text Extraction From Images
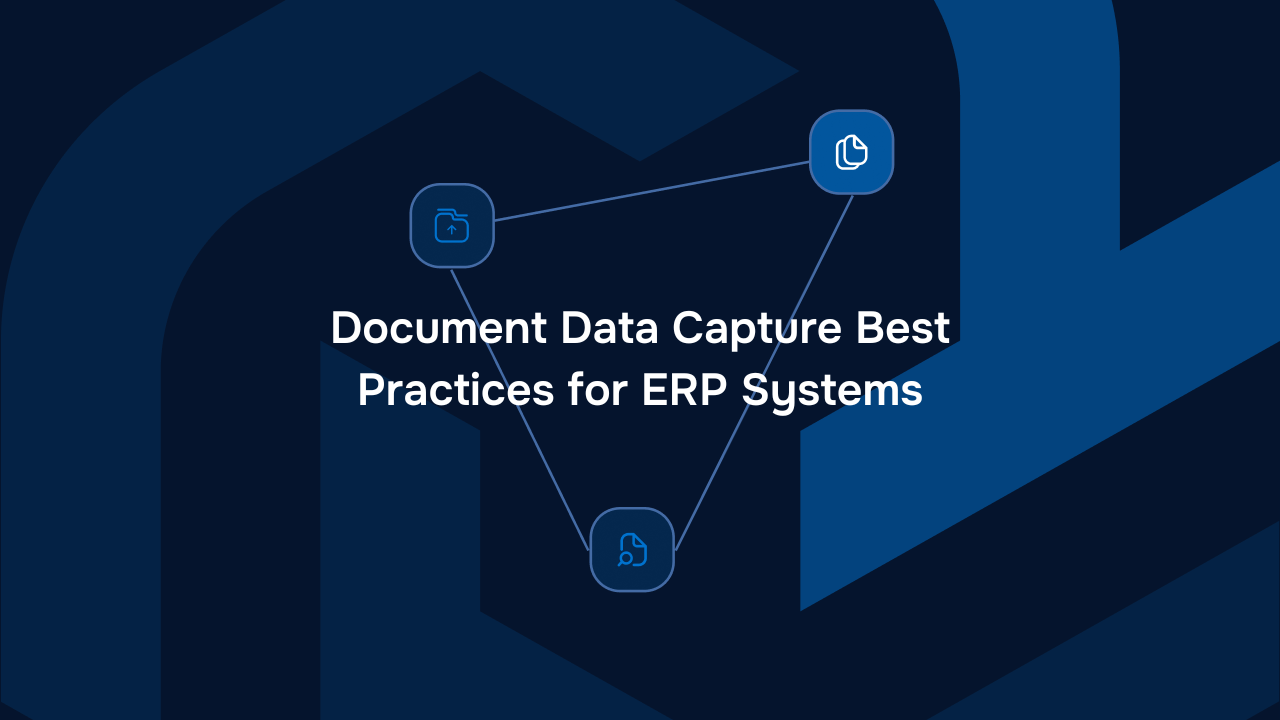
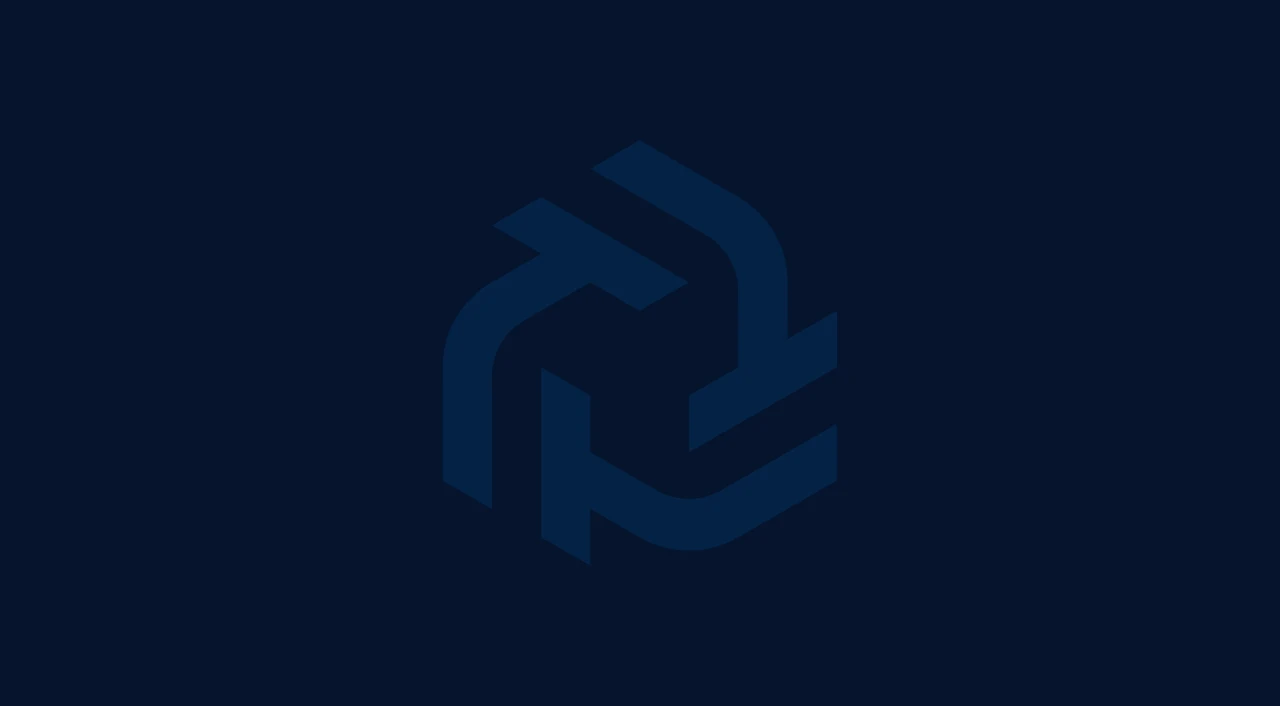